Appendix A: Kernel SVM and Kernel Regression#
Computer Science Dept, Ferdowsi University of Mashhad
I should mention that the original material of this course was from Open Machine Learning Course, by Joaquin Vanschoren and others.
Show code cell source
# Auto-setup when running on Google Colab
import os
if 'google.colab' in str(get_ipython()) and not os.path.exists('/content/machine-learning'):
!git clone -q https://github.com/fum-cs/machine-learning.git /content/machine-learning
!pip --quiet install -r /content/machine-learning/requirements_colab.txt
%cd machine-learning/notebooks
# Global imports and settings
%matplotlib inline
from preamble import *
interactive = True # Set to True for interactive plots
if interactive:
fig_scale = 0.5
plt.rcParams.update(print_config)
else: # For printing
fig_scale = 1.3
plt.rcParams.update(print_config)
Example: SVMs#
Linear SVMs (dual form, for \(l\) support vectors with dual coefficients \(a_i\) and classes \(y_i\)):
Kernelized SVM, using any existing kernel \(k\) we want:
Show code cell source
from sklearn import svm
def plot_svm_kernels(kernels, poly_degree=3, gamma=2, C=1, size=4):
# Our dataset and targets
X = np.c_[(.4, -.7),
(-1.5, -1),
(-1.4, -.9),
(-1.3, -1.2),
(-1.1, -.2),
(-1.2, -.4),
(-.5, 1.2),
(-1.5, 2.1),
(1, 1),
# --
(1.3, .8),
(1.2, .5),
(.2, -2),
(.5, -2.4),
(.2, -2.3),
(0, -2.7),
(1.3, 2.1)].T
Y = [0] * 8 + [1] * 8
# figure number
fig, axes = plt.subplots(-(-len(kernels)//3), min(len(kernels),3), figsize=(min(len(kernels),3)*size*1.2*fig_scale, -(-len(kernels)//3)*size*fig_scale), tight_layout=True)
if len(kernels) == 1:
axes = np.array([axes])
if not isinstance(gamma,list):
gamma = [gamma]*len(kernels)
if not isinstance(C,list):
C = [C]*len(kernels)
# fit the model
for kernel, ax, g, c in zip(kernels,axes.reshape(-1),gamma,C):
clf = svm.SVC(kernel=kernel, gamma=g, C=c, degree=poly_degree)
clf.fit(X, Y)
# plot the line, the points, and the nearest vectors to the plane
if kernel == 'rbf':
ax.set_title(r"kernel = {}, $\gamma$={}, C={}".format(kernel, g, c), pad=0.1)
else:
ax.set_title('kernel = %s' % kernel,pad=0.1)
ax.scatter(clf.support_vectors_[:, 0], clf.support_vectors_[:, 1],
s=25, edgecolors='grey', c='w', zorder=10, linewidths=0.5)
ax.scatter(X[:, 0], X[:, 1], c=Y, zorder=10, cmap=plt.cm.bwr, s=10*fig_scale)
for i, coef in enumerate(clf.dual_coef_[0]):
ax.annotate("%0.2f" % (coef), (clf.support_vectors_[i, 0]+0.1,clf.support_vectors_[i, 1]+0.25), zorder=11, fontsize=3)
ax.axis('tight')
x_min = -3
x_max = 3
y_min = -3
y_max = 3
XX, YY = np.mgrid[x_min:x_max:200j, y_min:y_max:200j]
Z = clf.decision_function(np.c_[XX.ravel(), YY.ravel()])
# Put the result into a color plot
Z = Z.reshape(XX.shape)
#plt.pcolormesh(XX, YY, Z > 0, cmap=plt.cm.bwr, alpha=0.1)
ax.contour(XX, YY, Z, colors=['k', 'k', 'k', 'k', 'k'], linestyles=['--', ':', '-', ':', '--'],
levels=[-1, -0.5, 0, 0.5, 1])
ax.set_xlim(x_min, x_max)
ax.set_ylim(y_min, y_max)
ax.set_xticks([])
ax.set_yticks([])
plt.tight_layout()
plt.show()
plot_svm_kernels(['linear', 'poly'],poly_degree=3,size=3.5)
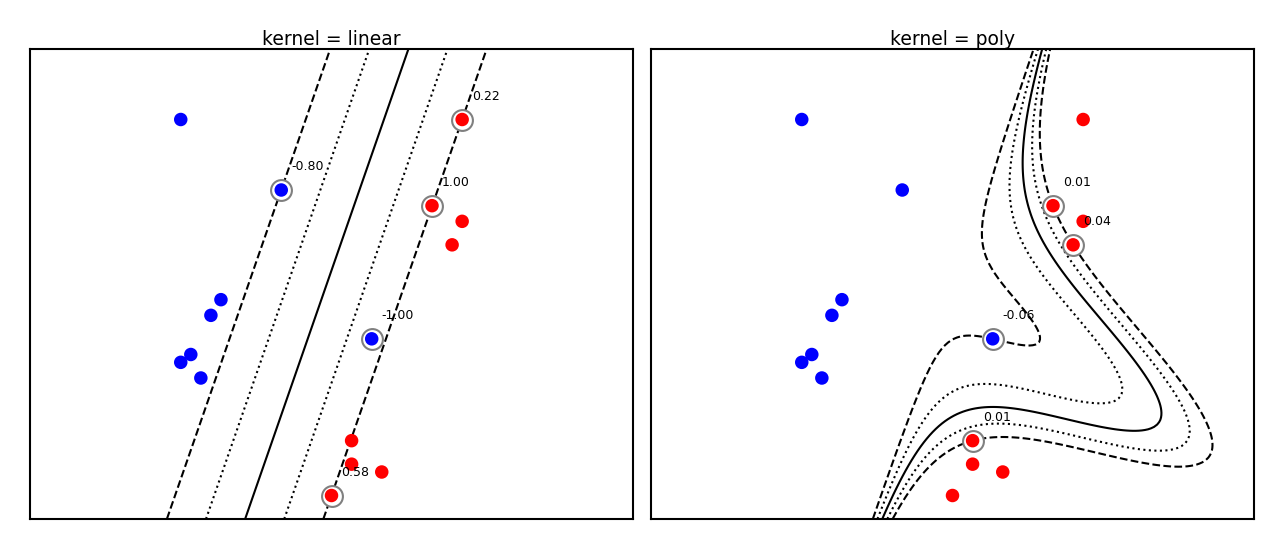
Which kernels exist?#
A (Mercer) kernel is any function \(k: X \times X \rightarrow \mathbb{R}\) with these properties:
Symmetry: \(k(\mathbf{x_1},\mathbf{x_2}) = k(\mathbf{x_2},\mathbf{x_1}) \,\,\, \forall \mathbf{x_1},\mathbf{x_2} \in X\)
Positive definite: the kernel matrix \(K\) is positive semi-definite
Intuitively, \(k(\mathbf{x_1},\mathbf{x_2}) \geq 0\)
The kernel matrix (or Gram matrix) for \(n\) points of \(x_1,..., x_n \in X\) is defined as:
Once computed (\(\mathcal{O}(n^2)\)), simply lookup \(k(\mathbf{x_1}, \mathbf{x_2})\) for any two points
In practice, you can either supply a kernel function or precompute the kernel matrix
Linear kernel#
Input space is same as output space: \(X = \mathcal{H} = \mathbb{R}^d\)
Feature map \(\Phi(\mathbf{x}) = \mathbf{x}\)
Kernel: \( k_{linear}(\mathbf{x_i},\mathbf{x_j}) = \mathbf{x_i} \cdot \mathbf{x_j}\)
Geometrically, the dot product is the projection of \(\mathbf{x_j}\) on hyperplane defined by \(\mathbf{x_i}\)
Becomes larger if \(\mathbf{x_i}\) and \(\mathbf{x_j}\) are in the same ‘direction’
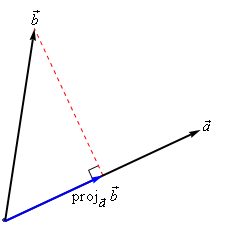
Linear kernel between point (0,1) and another unit vector an angle \(a\) (in radians)
Points with similar angles are deemed similar
Show code cell source
from sklearn.metrics.pairwise import linear_kernel, polynomial_kernel, rbf_kernel
import math
import ipywidgets as widgets
from ipywidgets import interact, interact_manual
def plot_lin_kernel():
fig, ax = plt.subplots(figsize=(5*fig_scale,3*fig_scale))
x = np.linspace(-math.pi,math.pi,100)
# compare point (0,1) with unit vector at certain angle
# linear_kernel returns the kernel matrix, get first element
ax.plot(x,[linear_kernel([[1,0]], [[math.cos(i),math.sin(i)]])[0] for i in x],lw=2*fig_scale,c='b',label='', linestyle='-')
ax.set_xlabel("angle $(-\pi,\pi)$ between unit vectors $x_1$ and $x_2$")
ax.set_ylabel("lin_kernel")
plt.grid()
plot_lin_kernel()
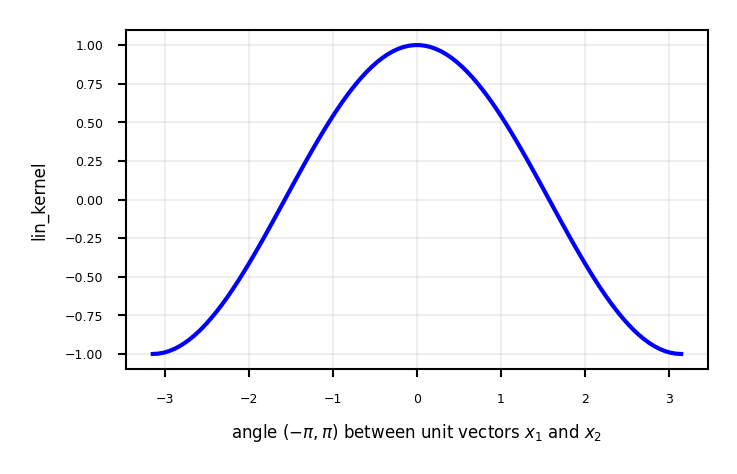
Polynomial kernel#
If \(k_1\), \(k_2\) are kernels, then \(\lambda . k_1\) (\(\lambda \geq 0\)), \(k_1 + k_2\), and \(k_1 . k_2\) are also kernels
The polynomial kernel (for degree \(d \in \mathbb{N}\)) reproduces the polynomial feature map
\(\gamma\) is a scaling hyperparameter (default \(\frac{1}{p}\))
\(c_0\) is a hyperparameter (default 1) to trade off influence of higher-order terms $\(k_{poly}(\mathbf{x_1},\mathbf{x_2}) = (\gamma (\mathbf{x_1} \cdot \mathbf{x_2}) + c_0)^d\)$
Show code cell source
@interact
def plot_poly_kernel(degree=(1,10,1), coef0=(0,2,0.5), gamma=(0,2,0.5)):
fig, ax = plt.subplots(figsize=(5*fig_scale,3*fig_scale))
x = np.linspace(-math.pi,math.pi,100)
# compare point (0,1) with unit vector at certain angle
if isinstance(degree,list):
for d in degree:
ax.plot(x,[polynomial_kernel([[1,0]], [[math.cos(i),math.sin(i)]], degree=d, coef0=coef0, gamma=gamma)[0]
for i in x],lw=2*fig_scale,label='degree = {}'.format(d), linestyle='-')
else:
ax.plot(x,[polynomial_kernel([[1,0]], [[math.cos(i),math.sin(i)]], degree=degree, coef0=coef0, gamma=gamma)[0]
for i in x],lw=2*fig_scale,c='b',label='degree = {}'.format(degree), linestyle='-')
ax.set_xlabel("angle $(-\pi,\pi)$ between unit vectors $x_1$ and $x_2$")
ax.set_ylabel("poly_kernel")
plt.grid()
plt.legend()
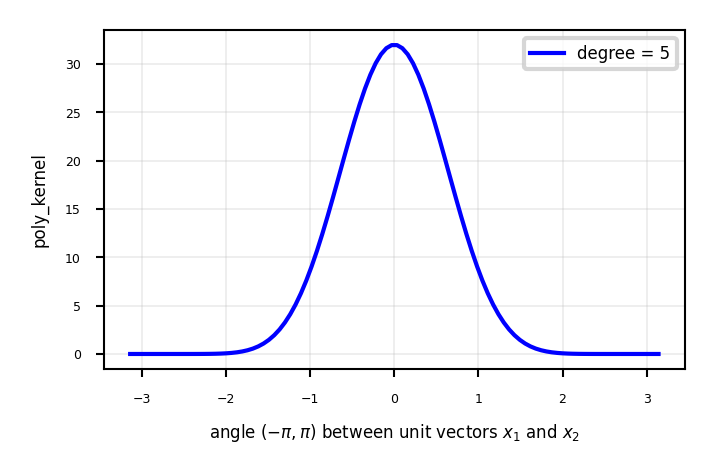
Show code cell source
if not interactive:
plot_poly_kernel(degree=[2,3,5], coef0=1, gamma=None)
RBF (Gaussian) kernel#
The Radial Basis Function (RBF) feature map is related to the Taylor series expansion of \(e^x\) $\(\Phi(x) = e^{-x^2/2\gamma^2} \Big[ 1, \sqrt{\frac{1}{1!\gamma^2}}x,\sqrt{\frac{1}{2!\gamma^4}}x^2,\sqrt{\frac{1}{3!\gamma^6}}x^3,\ldots\Big]^T\)$
RBF (or Gaussian ) kernel with kernel width \(\gamma \geq 0\):
$\(k_{RBF}(\mathbf{x_1},\mathbf{x_2}) = exp(-\gamma ||\mathbf{x_1} - \mathbf{x_2}||^2)\)$
Show code cell source
from sklearn.metrics.pairwise import polynomial_kernel, rbf_kernel
import ipywidgets as widgets
from ipywidgets import interact, interact_manual
@interact
def plot_rbf_kernel(gamma=(0.01,10,0.1)):
fig, ax = plt.subplots(figsize=(4*fig_scale,2.5*fig_scale))
x = np.linspace(-6,6,100)
if isinstance(gamma,list):
for g in gamma:
ax.plot(x,[rbf_kernel([[i]], [[0]], gamma=g)[0] for i in x],lw=2*fig_scale,label='gamma = {}'.format(g), linestyle='-')
else:
ax.plot(x,[rbf_kernel([[i]], [[0]], gamma=gamma)[0] for i in x],lw=2*fig_scale,c='b',label='gamma = {}'.format(gamma), linestyle='-')
ax.set_xlabel("x (distance )",labelpad=0.1)
ax.set_ylabel("rbf_kernel(0,x)")
ax.set_ylim(0,1)
ax.legend(bbox_to_anchor=(1.05, 1), loc=2)
plt.grid()
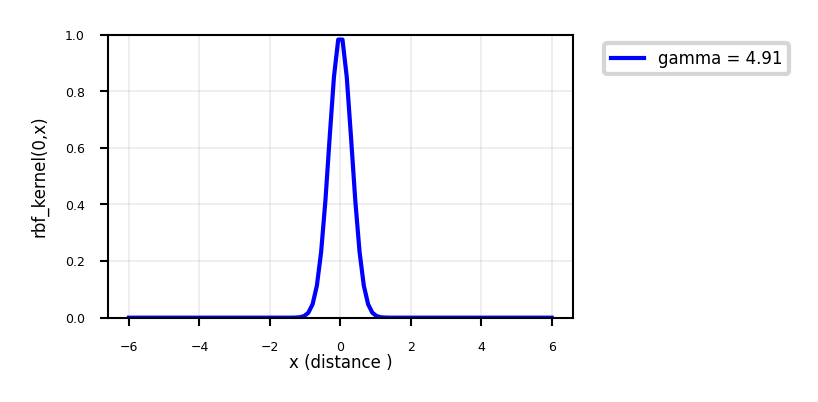
Taylor expansion: the more we add components, the more we can approximate any function
import matplotlib.cm as cm
# Generate x values
x = np.linspace(-2, 2, 100)
# Calculate corresponding y values for e^x
y_actual = np.exp(x)
# Plot the actual function
fig, ax = plt.subplots(figsize=(5*fig_scale,3*fig_scale))
plt.plot(x, y_actual, label='e^x', linewidth=1, color='black')
# Plot the individual terms of the Taylor expansion with formulas in the legend
colors = cm.gist_rainbow(np.linspace(0, 1, 10)) # Color scheme from red to blue
for n in range(10):
term = x**n / np.math.factorial(n)
partial_sum = np.sum([x**i / np.math.factorial(i) for i in range(n + 1)], axis=0)
# Plot partial sums with different colors
plt.plot(x, partial_sum, label=f'Partial Sum {n}', linestyle='dashed', color=colors[n])
# Add labels and title
plt.xlabel('x')
plt.ylabel('y')
plt.title('Components and Partial Sums of $e^x$ and its Taylor Expansion')
# Display legend
plt.legend();
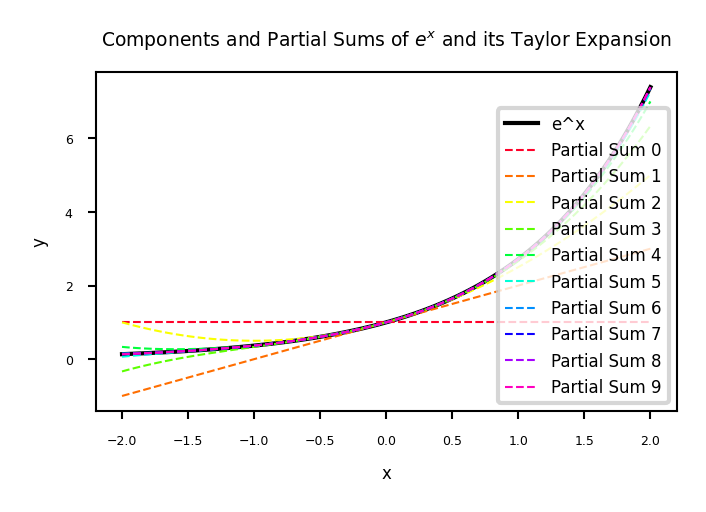
Show code cell source
if not interactive:
plot_rbf_kernel(gamma=[0.1,0.5,10])
The RBF kernel \(k_{RBF}(\mathbf{x_1},\mathbf{x_2}) = exp(-\gamma ||\mathbf{x_1} - \mathbf{x_2}||^2)\) does not use a dot product
It only considers the distance between \(\mathbf{x_1}\) and \(\mathbf{x_2}\)
It’s a local kernel : every data point only influences data points nearby
linear and polynomial kernels are global : every point affects the whole space
Similarity depends on closeness of points and kernel width
value goes up for closer points and wider kernels (larger overlap)
Show code cell source
def gaussian(x, mu, gamma):
k=2 # Doubling the distance makes the figure more interpretable
return np.exp(-gamma * np.power((x - mu)*k, 2.))
@interact
def plot_rbf_kernel_value(gamma=(0.1,1,0.1),x_1=(-6,6,0.1),x_2=(-6,6,0.1)):
fig, ax = plt.subplots(figsize=(6*fig_scale,4*fig_scale))
x = np.linspace(-6,6,100)
ax.plot(x,gaussian(x, x_1, gamma),lw=2*fig_scale,label='gaussian at x_1'.format(gamma), linestyle='-')
ax.plot(x,gaussian(x, x_2, gamma),lw=2*fig_scale,label='gaussian at x_2'.format(gamma), linestyle='-')
ax.plot(x,[rbf_kernel([[x_1]], [[x_2]], gamma=gamma)[0]]*len(x),lw=2,c='g',label='rfb_kernel(x_1,x_2)', linestyle='-')
ax.set_xlabel("x")
ax.set_ylabel(r"rbf_kernel($\gamma$={})".format(gamma))
ax.set_ylim(0,1)
ax.legend(bbox_to_anchor=(1.05, 1), loc=2)
plt.grid()
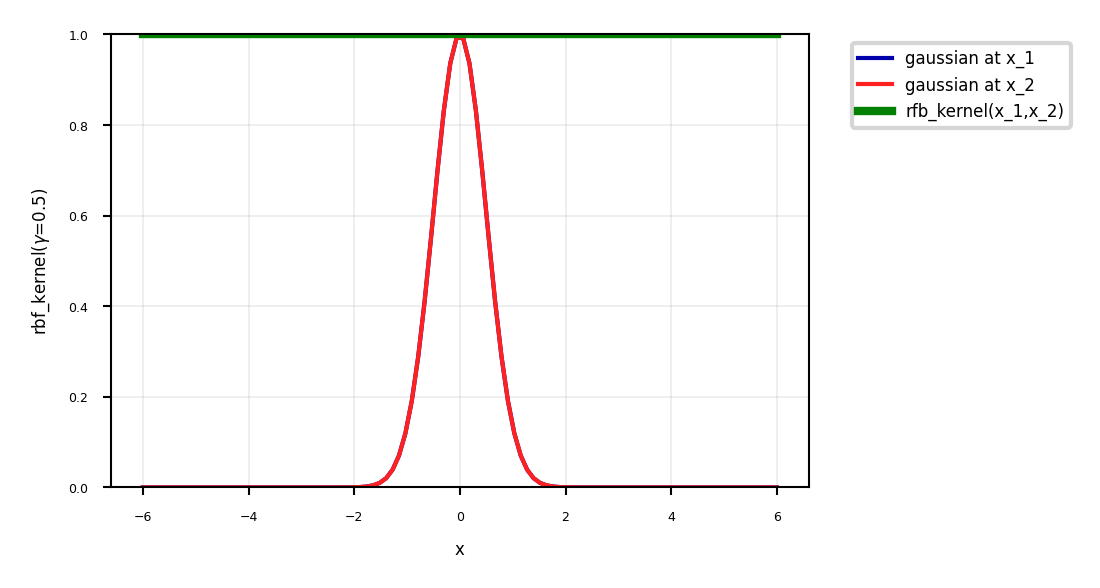
Show code cell source
if not interactive:
plot_rbf_kernel_value(gamma=0.2,x_1=0,x_2=2)
Kernelized SVMs in practice#
You can use SVMs with any kernel to learn non-linear decision boundaries
Show code cell source
plot_svm_kernels(['linear', 'poly', 'rbf'],poly_degree=3,gamma=2,size=4)
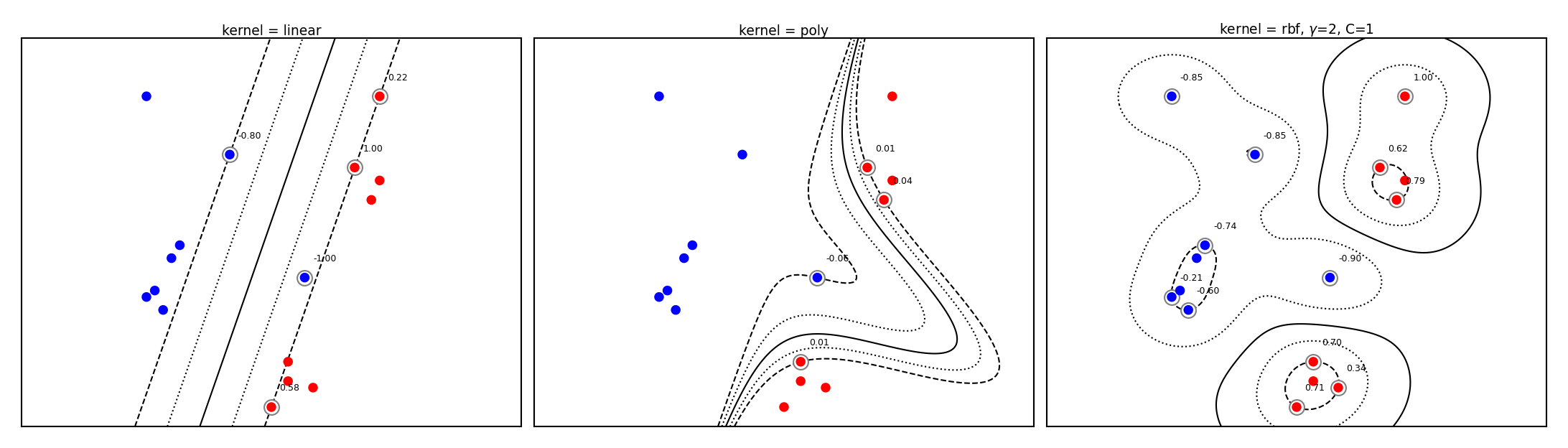
SVM with RBF kernel#
Every support vector locally influences predictions, according to kernel width (\(\gamma\))
The prediction for test point \(\mathbf{u}\): sum of the remaining influence of each support vector
\(f(x) = \sum_{i=1}^{l} a_i y_i k(\mathbf{x_i},\mathbf{u})\)
Show code cell source
@interact
def plot_rbf_data(gamma=(0.1,10,0.5),C=(0.01,5,0.1)):
plot_svm_kernels(['rbf'],gamma=gamma,C=C,size=5)
Show code cell source
if not interactive:
plot_svm_kernels(['rbf','rbf','rbf'],gamma=[0.1,1,5],size=4)
Tuning RBF SVMs#
gamma (kernel width)
high values cause narrow Gaussians, more support vectors, overfitting
low values cause wide Gaussians, underfitting
C (cost of margin violations)
high values punish margin violations, cause narrow margins, overfitting
low values cause wider margins, more support vectors, underfitting
Show code cell source
%%HTML
<style>
.reveal img {
margin-top: 0px;
}
</style>
Show code cell source
plot_svm_kernels(['rbf','rbf','rbf','rbf','rbf','rbf','rbf','rbf','rbf'],
gamma=[0.1,1,5,0.1,1,5,0.1,1,5],
C=[0.001,0.001,0.001,1,1,1,100,100,100],size=2.6)
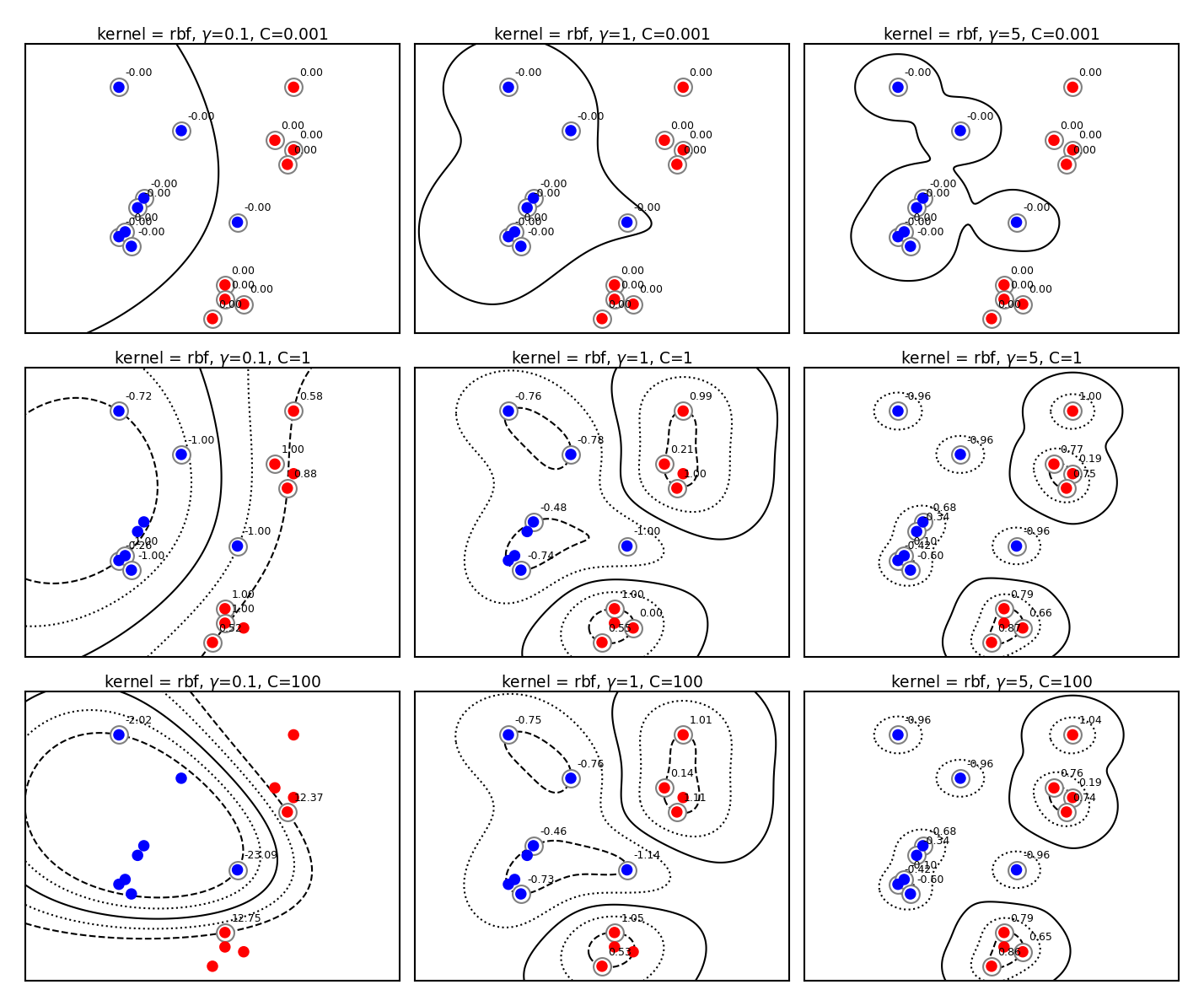
Kernel overview
Show code cell source
from sklearn.svm import SVC
names = ["Linear SVM", "RBF", "Polynomial"]
classifiers = [
SVC(kernel="linear", C=0.025),
SVC(gamma=2, C=1),
SVC(kernel="poly", degree=3, C=0.1)
]
mglearn.plots.plot_classifiers(names, classifiers, figuresize=(20*fig_scale,8*fig_scale))
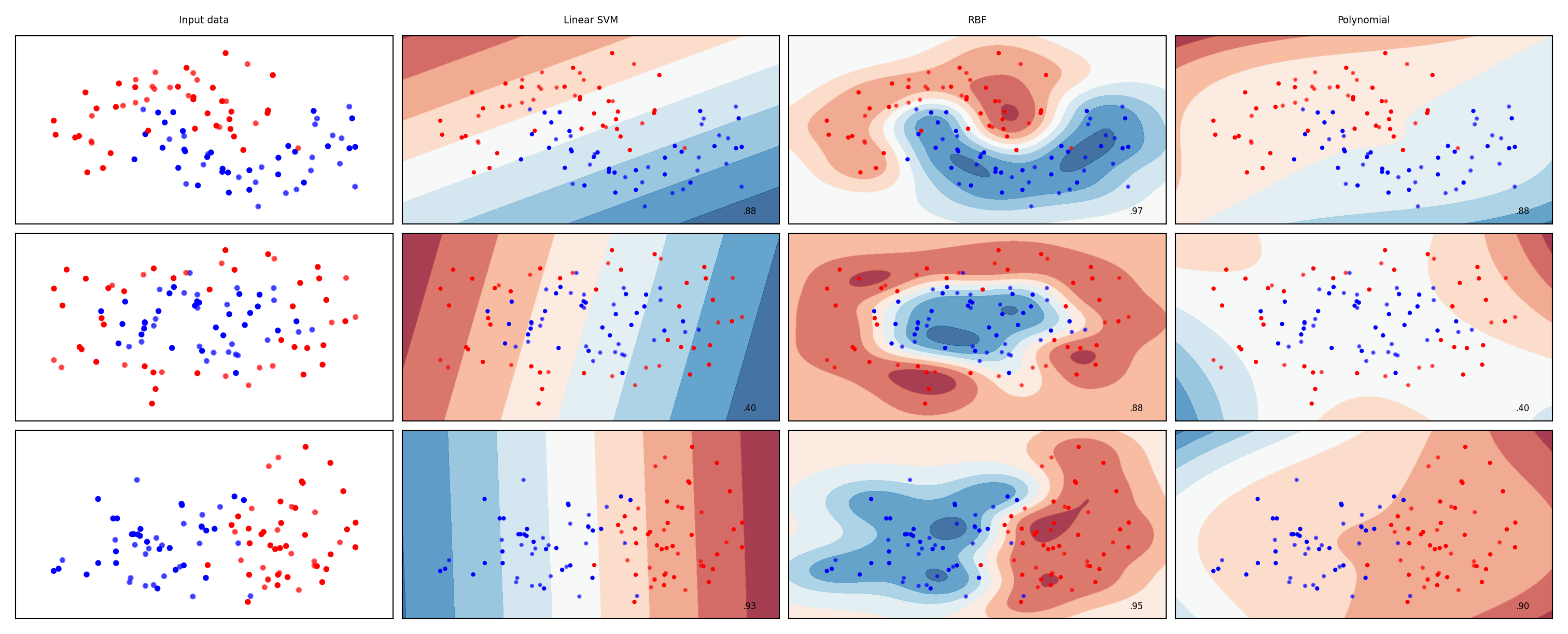
SVMs in practice#
C and gamma always need to be tuned
Interacting regularizers. Find a good C, then finetune gamma
SVMs expect all features to be approximately on the same scale
Data needs to be scaled beforehand
Allow to learn complex decision boundaries, even with few features
Work well on both low- and high dimensional data
Especially good at small, high-dimensional data
Hard to inspect, although support vectors can be inspected
In sklearn, you can use
SVC
for classification with a range of kernelsSVR
for regression
Other kernels#
There are many more possible kernels
If no kernel function exists, we can still precompute the kernel matrix
All you need is some similarity measure, and you can use SVMs
Text kernels:
Word kernels: build a bag-of-words representation of the text (e.g. TFIDF)
Kernel is the inner product between these vectors
Subsequence kernels: sequences are similar if they share many sub-sequences
Build a kernel matrix based on pairwise similarities
Graph kernels: Same idea (e.g. find common subgraphs to measure similarity)
These days, deep learning embeddings are more frequently used
The Representer Theorem#
We can kernelize many other loss functions as well
The Representer Theorem states that if we have a loss function \(\mathcal{L}'\) with
\(\mathcal{L}\) an arbitrary loss function using some function \(f\) of the inputs \(\mathbf{x}\)
\(\mathcal{R}\) a (non-decreasing) regularization score (e.g. L1 or L2) and constant \(\lambda\) $\(\mathcal{\mathcal{L}'}(\mathbf{w}) = \mathcal{L}(y,f(\mathbf{x})) + \lambda \mathcal{R} (||\mathbf{w}||)\)$
Then the weights \(\mathbf{w}\) can be described as a linear combination of the training samples: $\(\mathbf{w} = \sum_{i=1}^{n} a_i y_i f(\mathbf{x_i})\)$
Note that this is exactly what we found for SVMs: \( \mathbf{w} = \sum_{i=1}^{l} a_i y_i \mathbf{\mathbf{x_i}} \)
Hence, we can also kernelize Ridge regression, Logistic regression, Perceptrons, Support Vector Regression, …
Kernelized Ridge regression#
The linear Ridge regression loss (with \(\mathbf{x_0}=1\)): $\(\mathcal{L}_{Ridge}(\mathbf{w}) = \sum_{i=0}^{n} (y_i-\mathbf{w}\mathbf{x_i})^2 + \lambda \| w \|^2\)$
Filling in \(\mathbf{w} = \sum_{i=1}^{n} \alpha_i y_i \mathbf{x_i}\) yields the dual formulation: $\(\mathcal{L}_{Ridge}(\mathbf{w}) = \sum_{i=1}^{n} (y_i-\sum_{j=1}^{n} \alpha_j y_j \mathbf{x_i}\mathbf{x_j})^2 + \lambda \sum_{i=1}^{n} \sum_{j=1}^{n} \alpha_i \alpha_j y_i y_j \mathbf{x_i}\mathbf{x_j}\)$
Generalize \(\mathbf{x_i}\cdot\mathbf{x_j}\) to \(k(\mathbf{x_i},\mathbf{x_j})\) $\(\mathcal{L}_{KernelRidge}(\mathbf{\alpha},k) = \sum_{i=1}^{n} (y_i-\sum_{j=1}^{n} \alpha_j y_j k(\mathbf{x_i},\mathbf{x_n}))^2 + \lambda \sum_{i=1}^{n} \sum_{j=1}^{n} \alpha_i \alpha_j y_i y_j k(\mathbf{x_i},\mathbf{x_j})\)$
Example of kernelized Ridge#
Prediction (red) is now a linear combination of kernels (blue): \(y = \sum_{j=1}^{n} \alpha_j y_j k(\mathbf{x},\mathbf{x_j})\)
We learn a dual coefficient for each point
Show code cell source
import scipy.stats as stats
import math
@interact
def plot_kernel_ridge(gamma=(0.1,2,0.1),a1=(-1,1,0.1),a2=(-1,1,0.1),a3=(-1,1,0.1)):
fig, ax = plt.subplots(figsize=(10*fig_scale,5*fig_scale))
xs = [-6,-4,-3,0,2,4]
ys = [-0.3,0.05,-0.1,0.3,0.16,-0.05]
alphas = [a1,a2,a3,.7,0.3,-0.2]
p = np.linspace(-10, 10, 100)
f = [0]*100
for a,x,y in zip(alphas,xs,ys):
k = stats.norm.pdf(p, x, 1/gamma)
f += a*k
plt.plot(p, k, 'b-', lw=1*fig_scale)
plt.annotate("%0.2f" % (a), (x+0.1,y+0.01), fontsize=20*fig_scale, zorder=11)
plt.plot(p, f, 'r-', lw=3*fig_scale)
plt.scatter(xs,ys);
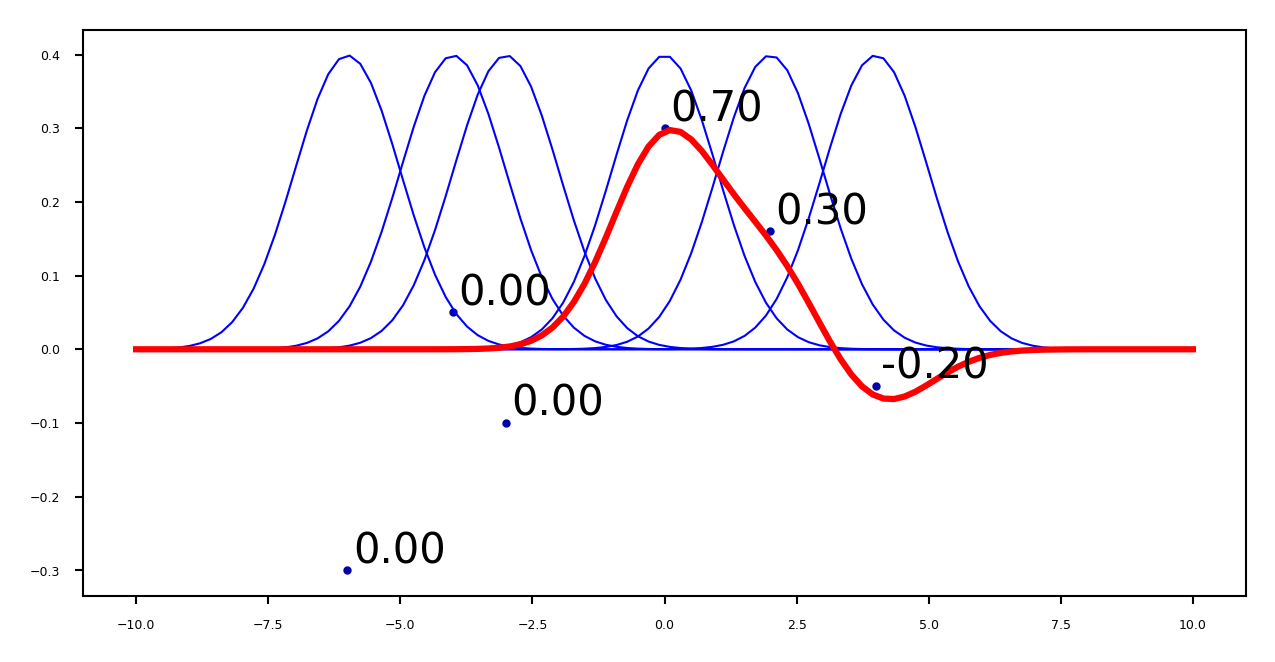
Show code cell source
if not interactive:
plot_kernel_ridge(1,-.8,.5,-0.5)
Fitting our regression data with
KernelRidge
Show code cell source
from sklearn.kernel_ridge import KernelRidge
@interact
def plot_kernel_ridge(gamma=(0.01,10,0.5)):
X, y = mglearn.datasets.make_wave(n_samples=100)
line = np.linspace(-3, 3, 1000, endpoint=False).reshape(-1, 1)
reg = KernelRidge(kernel='rbf', gamma=gamma).fit(X, y)
fig = plt.figure(figsize=(8*fig_scale,4*fig_scale))
plt.plot(line, reg.predict(line), label="Kernel Ridge (RBF)", lw=2*fig_scale, c='r')
plt.plot(X[:, 0], y, 'o', c='k')
plt.ylabel("Regression output")
plt.xlabel("Input feature")
plt.legend(loc="best");
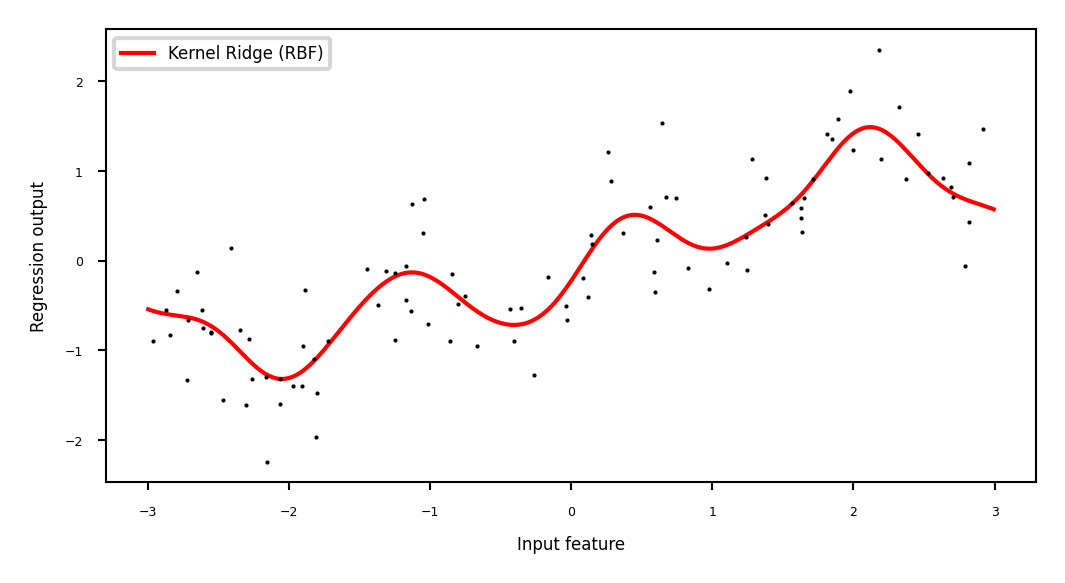
Show code cell source
if not interactive:
plot_kernel_ridge(5)
Other kernelized methods#
Same procedure can be done for logistic regression
For perceptrons, \(\alpha \rightarrow \alpha+1\) after every misclassification $\(\mathcal{L}_{DualPerceptron}(x_i,k) = max(0,y_i \sum_{j=1}^{n} \alpha_j y_j k(\mathbf{x_j},\mathbf{x_i}))\)$
Support Vector Regression behaves similarly to Kernel Ridge
Show code cell source
from sklearn.svm import SVR
@interact
def plot_kernel_ridge_svr(gamma=(0.01,10,0.5)):
X, y = mglearn.datasets.make_wave(n_samples=100)
line = np.linspace(-3, 3, 1000, endpoint=False).reshape(-1, 1)
reg = KernelRidge(kernel='rbf', gamma=gamma).fit(X, y)
svr = SVR(kernel='rbf', gamma=gamma).fit(X, y)
fig = plt.figure(figsize=(8*fig_scale,4*fig_scale))
plt.plot(line, reg.predict(line), label="Kernel Ridge (RBF)", lw=2*fig_scale, c='r')
plt.plot(line, svr.predict(line), label="Support Vector Regression (RBF)", lw=2*fig_scale, c='b')
plt.plot(X[:, 0], y, 'o', c='k')
plt.ylabel("Regression output")
plt.xlabel("Input feature")
plt.legend(loc="best");
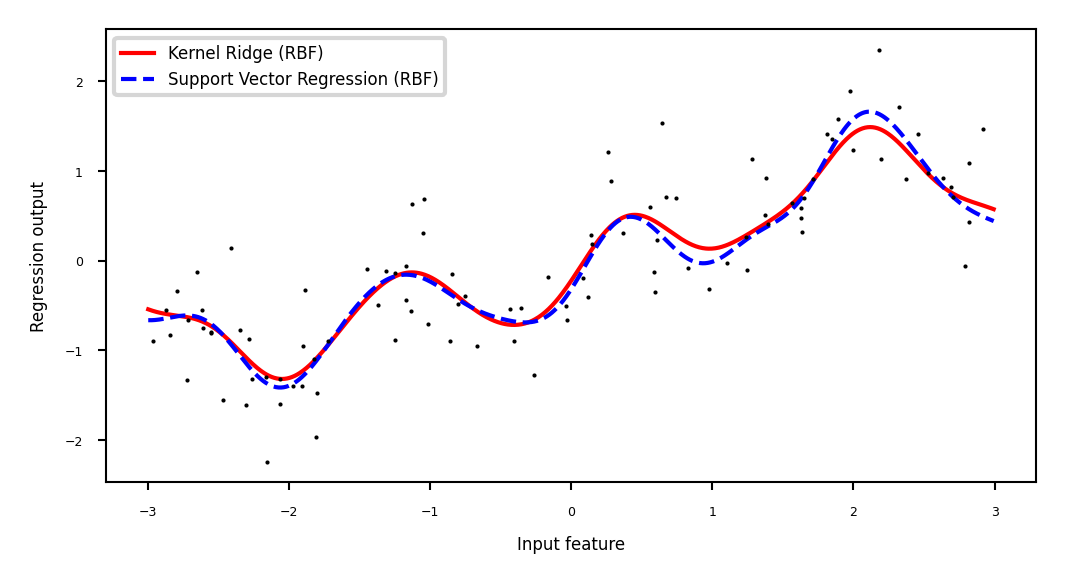
Show code cell source
if not interactive:
plot_kernel_ridge_svr(gamma=5)
Summary#
Feature maps \(\Phi(x)\) transform features to create a higher-dimensional space
Allows learning non-linear functions or boundaries, but very expensive/slow
For some \(\Phi(x)\), we can compute dot products without constructing this space
Kernel trick: \(k(\mathbf{x_i},\mathbf{x_j}) = \Phi(\mathbf{x_i}) \cdot \Phi(\mathbf{x_j})\)
Kernel \(k\) (generalized dot product) is a measure of similarity between \(\mathbf{x_i}\) and \(\mathbf{x_j}\)
There are many such kernels
Polynomial kernel: \(k_{poly}(\mathbf{x_1},\mathbf{x_2}) = (\gamma (\mathbf{x_1} \cdot \mathbf{x_2}) + c_0)^d\)
RBF (Gaussian) kernel: \(k_{RBF}(\mathbf{x_1},\mathbf{x_2}) = exp(-\gamma ||\mathbf{x_1} - \mathbf{x_2}||^2)\)
A kernel matrix can be precomputed using any similarity measure (e.g. for text, graphs,…)
Any loss function where inputs appear only as dot products can be kernelized
E.g. Linear SVMs: simply replace the dot product with a kernel of choice
The Representer theorem states which other loss functions can also be kernelized and how
Ridge regression, Logistic regression, Perceptrons,…