Lecture 7. Bayesian Learning#
Learning in an uncertain world
Mahmood Amintoosi, Spring 2024
Computer Science Dept, Ferdowsi University of Mashhad
I should mention that the original material of this course was from Open Machine Learning Course, by Joaquin Vanschoren and others.
Show code cell source
# Auto-setup when running on Google Colab
import os
if 'google.colab' in str(get_ipython()) and not os.path.exists('/content/machine-learning'):
!git clone -q https://github.com/fum-cs/machine-learning.git /content/machine-learning
!pip --quiet install -r /content/machine-learning/requirements_colab.txt
%cd machine-learning/notebooks
# Global imports and settings
%matplotlib inline
from preamble import *
interactive = False # Set to True for interactive plots
if interactive:
fig_scale = 0.61
print_config['font.size'] = 7
print_config['xtick.labelsize'] = 5
print_config['ytick.labelsize'] = 5
plt.rcParams.update(print_config)
else: # For printing
fig_scale = 0.28
plt.rcParams.update(print_config)
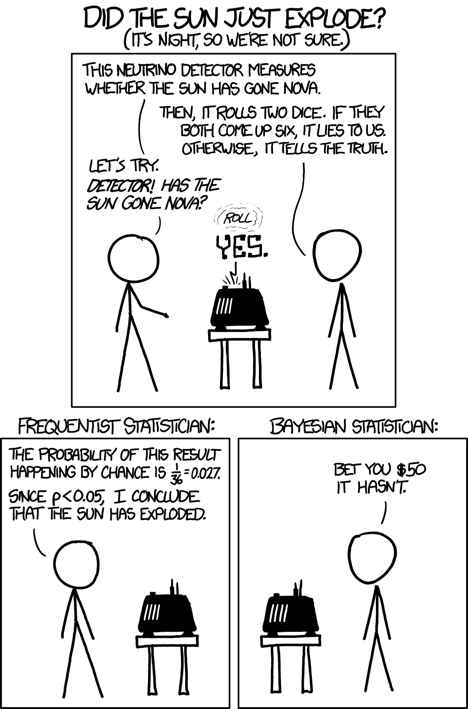
Bayes’ rule#
Rule for updating the probability of a hypothesis \(c\) given data \(x\)
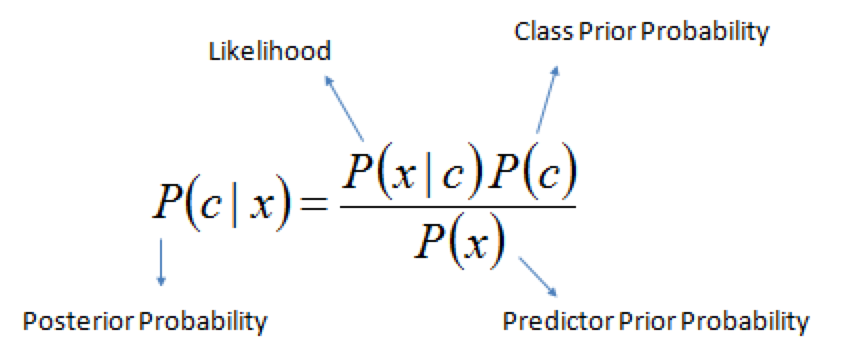
\(P(c|x)\) is the posterior probability of class \(c\) given data \(x\).
\(P(c)\) is the prior probability of class \(c\): what you believed before you saw the data \(x\)
\(P(x|c)\) is the likelihood of data point \(x\) given that the class is \(c\) (computed from your data)
\(P(x)\) is the prior probability of the data (marginal likelihood): the likelihood of the data \(x\) under any circumstance (no matter what the class is)
Bayesian models#
Learn the joint distribution \(P(x,y)=P(x|y)P(y)\).
Assumes that the data is Gaussian distributed (!)
With every input \(x\) you get \(P(y|x)\), hence a mean and standard deviation for \(y\) (blue)
For every desired output \(y\) you get \(P(x|y)\), hence you can sample new points \(x\) (red)
Easily updatable with new data using Bayes’ rule (‘turning the crank’)
Previous posterior \(P(y|x)\) becomes new prior \(P(y)\)
Show code cell source
import scipy.stats as stats
from matplotlib import cm
import matplotlib.pyplot as plt
from mpl_toolkits.mplot3d import axes3d
import ipywidgets as widgets
from ipywidgets import interact, interact_manual
def plot_joint_distribution(mean,covariance_matrix,x,y,contour=False,labels=['x','y'], plot_intersect=False, ax=None):
delta = 0.05
xr, yr = np.arange(-3.0, 3.0, delta), np.arange(-3.0, 3.0, delta)
X, Y = np.meshgrid(xr,yr)
xy = np.hstack((X.flatten()[:, None], Y.flatten()[:, None]))
p = stats.multivariate_normal.pdf(xy, mean=mean, cov=covariance_matrix)
Z = p.reshape(len(X), len(X))
if not ax:
fig = plt.figure(figsize=plt.figaspect(1)*fig_scale*1.1)
ax = fig.add_subplot(projection='3d')
if contour:
ax.plot_surface(X, Y, Z*0.001, alpha=0.9, cmap='jet')
else:
ax.plot_surface(X, Y, Z, alpha=0.9, cmap='jet')
cset = ax.contour(X, Y, Z, zdir='y', offset=3, cmap='Reds')
cset = ax.contour(X, Y, Z, zdir='x', offset=-3, cmap='Blues')
Zys = np.zeros_like(Z)
Zys[60,:] = Z[int((y+3)/delta)]
cset = ax.contour(X, Y, Zys, zdir='y', offset=3, linewidths=1.5, cmap='Reds')
Zys = np.zeros_like(Z)
Zys[:,60] = Z[int((x+3)/delta)]
cset = ax.contour(X, Y, Zys, zdir='x', offset=-3, linewidths=1.5, cmap='Blues')
if plot_intersect:
ax.plot([x]*len(xr), yr, 0.001, color='k', alpha=1, ls='-', lw=1)
ax.plot(xr, [y]*len(yr), 0.001, color='k', alpha=1, ls='-', lw=1)
ax.set_xlabel(labels[0], labelpad=-2/fig_scale)
ax.set_ylabel(labels[1], labelpad=-2/fig_scale)
ax.set_zlabel('Probability density', labelpad=-3/fig_scale)
ax.set_xticks([-3,0,3])
ax.set_yticks([-3,0,3])
ax.set_xlim(xmin=-3, xmax=3)
ax.set_ylim(ymin=-3, ymax=3)
#ax.tick_params(axis='both')
ax.set_zticks([])
ax.tick_params(axis='both', width=0, labelsize=10*fig_scale, pad=-3)
@interact
def interact_joint_distribution(x=(-3,3,0.5),y=(-3,3,0.5),contour=False):
# Shape of the joint distribution
mean = [0, 0]
covariance_matrix = np.array([[1,-0.8],[-0.8,0.8]])
plot_joint_distribution(mean,covariance_matrix,x,y,contour, plot_intersect=True)
Show code cell source
if not interactive:
mean = [0, 0]
covariance_matrix = np.array([[1,-0.8],[-0.8,1]])
fig = plt.figure(figsize=plt.figaspect(0.3)*fig_scale*2)
ax1 = fig.add_subplot(1, 2, 1, projection='3d')
plot_joint_distribution(mean,covariance_matrix,x=1,y=1,contour=False, plot_intersect=True, ax=ax1)
ax2 = fig.add_subplot(1, 2, 2, projection='3d')
plot_joint_distribution(mean,covariance_matrix,x=-1,y=1,contour=False, plot_intersect=True, ax=ax2)
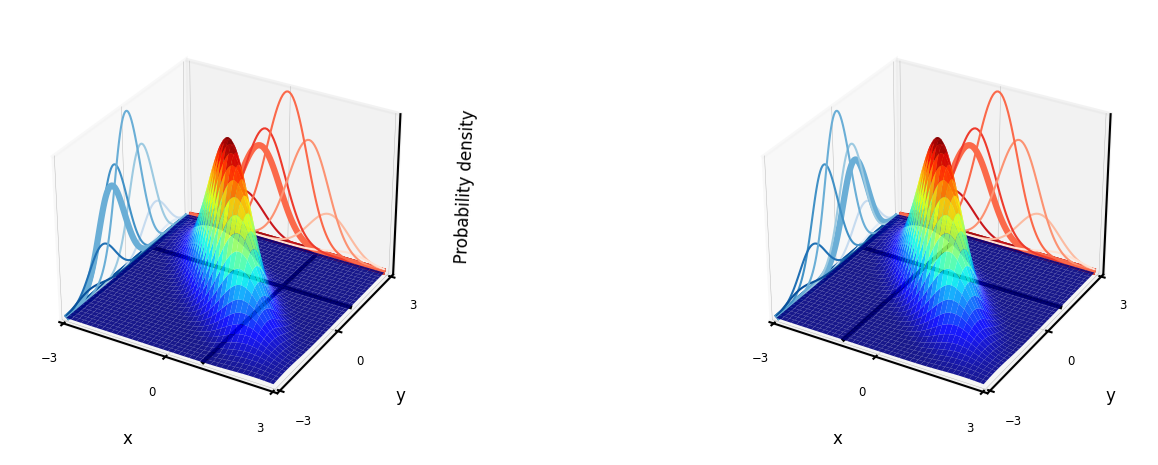
Generative models#
The joint distribution represents the training data for a particular output (e.g. a class)
You can sample a new point \(\textbf{x}\) with high predicted likelihood \(P(x,c)\): that new point will be very similar to the training points
Generate new (likely) points according to the same distribution: generative model
Generate examples that are fake but corresponding to a desired output
Generative neural networks (e.g. GANs) can do this very accurately for text, images, …
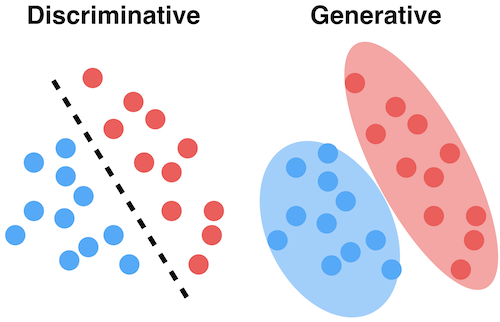
Naive Bayes#
Predict the probability that a point belongs to a certain class, using Bayes’ Theorem
Problem: since \(\textbf{x}\) is a vector, computing \(P(\textbf{x}|c)\) can be very complex
Naively assume that all features are conditionally independent from each other, in which case:
\(P(\mathbf{x}|c) = P(x_1|c) \times P(x_2|c) \times ... \times P(x_n|c)\)Very fast: only needs to extract statistics from each feature.
On categorical data#
What’s the probability that your friend will play golf if the weather is sunny?
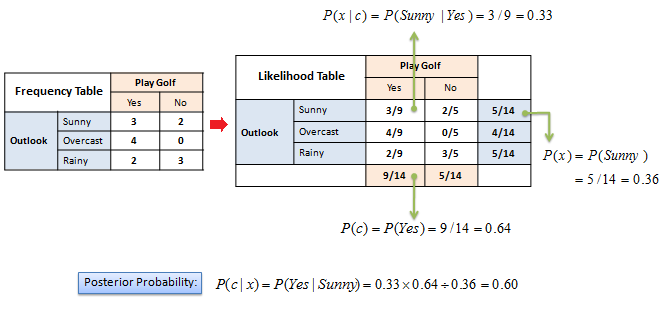
On numeric data#
We need to fit a distribution (e.g. Gaussian) over the data points
GaussianNB: Computes mean \(\mu_c\) and standard deviation \(\sigma_c\) of the feature values per class: \(p(x=v \mid c)=\frac{1}{\sqrt{2\pi\sigma^2_c}}\,e^{ -\frac{(v-\mu_c)^2}{2\sigma^2_c} }\)
We can now make predictions using Bayes’ theorem: \(p(c \mid \mathbf{x}) = \frac{p(\mathbf{x} \mid c) \ p(c)}{p(\mathbf{x})}\)
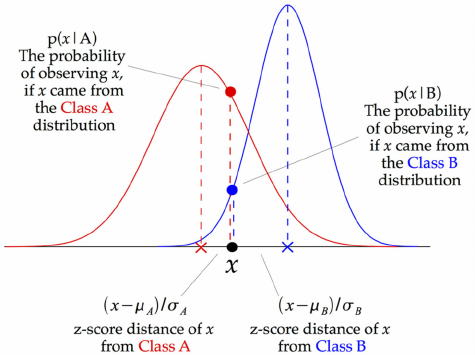
What do the predictions of Gaussian Naive Bayes look like?
Show code cell source
from sklearn.naive_bayes import GaussianNB
names = ["Naive Bayes"]
classifiers = [GaussianNB()]
mglearn.plots.plot_classifiers(names, classifiers, figuresize=(8*fig_scale,6*fig_scale))
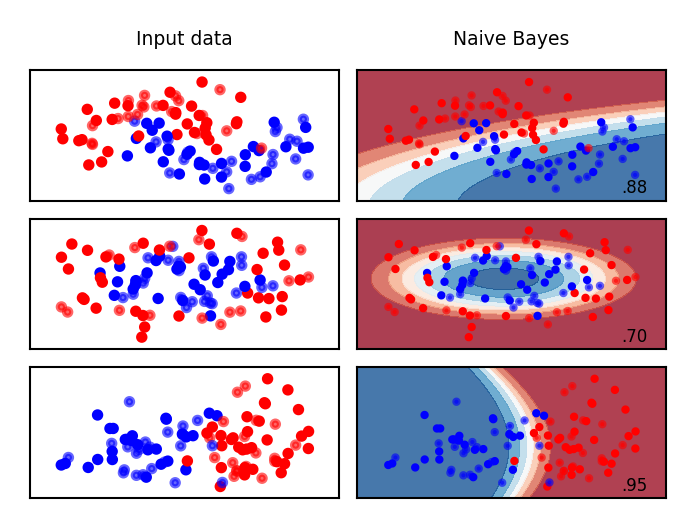
Other Naive Bayes classifiers:
BernoulliNB
Assumes binary data
Feature statistics: Number of non-zero entries per class
MultinomialNB
Assumes count data
Feature statistics: Average value per class
Mostly used for text classification (bag-of-words data)
Bayesian Networks#
What if we know that some variables are not independent?
A Bayesian Network is a directed acyclic graph representing variables as nodes and conditional dependencies as edges.
If an edge \((A, B)\) connects random variables A and B, then \(P(B|A)\) is a factor in the joint probability distribution. We must know \(P(B|A)\) for all values of \(B\) and \(A\)
The graph structure can be designed manually or learned (hard!)
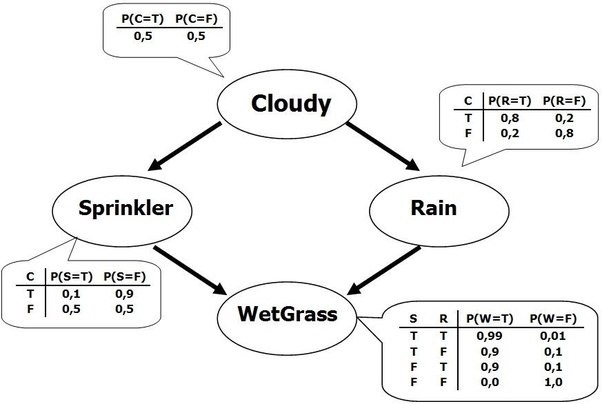
Show code cell source
### GP implementation (based on example by Neil Lawrence http://inverseprobability.com/mlai2015/)
# Compute covariances
def compute_kernel(X, X2, kernel, **kwargs):
K = np.zeros((X.shape[0], X2.shape[0]))
for i in np.arange(X.shape[0]):
for j in np.arange(X2.shape[0]):
K[i, j] = kernel(X[i, :], X2[j, :], **kwargs)
return K
# Exponentiated quadratic kernel (RBF)
def exponentiated_quadratic(x, x_prime, variance, lengthscale):
squared_distance = ((x-x_prime)**2).sum()
return variance*np.exp((-0.5*squared_distance)/lengthscale**2)
class GP():
def __init__(self, X, y, sigma2, kernel, **kwargs):
self.K = compute_kernel(X, X, kernel, **kwargs)
self.X = X
self.y = y
self.sigma2 = sigma2
self.kernel = kernel
self.kernel_args = kwargs
self.update_inverse()
def update_inverse(self):
# Precompute the inverse covariance and some quantities of interest
## NOTE: Not the correct *numerical* way to compute this! For ease of use.
self.Kinv = np.linalg.inv(self.K+self.sigma2*np.eye(self.K.shape[0]))
# the log determinant of the covariance matrix.
self.logdetK = np.linalg.det(self.K+self.sigma2*np.eye(self.K.shape[0]))
# The matrix inner product of the inverse covariance
self.Kinvy = np.dot(self.Kinv, self.y)
self.yKinvy = (self.y*self.Kinvy).sum()
def log_likelihood(self):
# use the pre-computes to return the likelihood
return -0.5*(self.K.shape[0]*np.log(2*np.pi) + self.logdetK + self.yKinvy)
def objective(self):
# use the pre-computes to return the objective function
return -self.log_likelihood()
def posterior_f(self, X_test, y):
K_star = compute_kernel(self.X, X_test, self.kernel, **self.kernel_args)
K_starstar = compute_kernel(X_test, X_test, self.kernel, **self.kernel_args)
A = np.dot(self.Kinv, K_star)
mu_f = np.dot(A.T, y)
C_f = K_starstar - np.dot(A.T, K_star)
return mu_f, C_f
# set covariance function parameters
variance = 16.0
lengthscale = 32
sigma2 = 0.05 # noise variance
# Plotting how GPs sample
def plot_gp(X, Y, X_test, nr_points=10, variance=16, lengthscale=32, add_mean=True, nr_samples=25, show_covariance=True, show_stdev=False, ylim=None):
# Compute GP
xs, ys = X[:nr_points], Y[:nr_points]
model = GP(xs, ys, sigma2, exponentiated_quadratic, variance=variance, lengthscale=lengthscale)
mu_f, C_f = model.posterior_f(X_test,ys)
# Plot GP with or without covariance matrix
if show_covariance:
fig, axs = plt.subplots(1, 2, figsize=(10*fig_scale, 4*fig_scale))
gp_axs = axs[0]
else:
fig, axs = plt.subplots(1, 1, figsize=(10*fig_scale, 4*fig_scale))
gp_axs = axs
# Plot GP
if add_mean:
samples = np.random.multivariate_normal(mu_f.T[0], C_f, nr_samples).T;
else:
samples = np.random.multivariate_normal(np.zeros(100), C_f, nr_samples).T;
if not show_stdev:
for i in range(samples.shape[1]):
gp_axs.plot(X_test, samples[:,i], c='b', ls='-', alpha=0.1);
gp_axs.plot(X_test, mu_f, lw=1, c='k', ls='-', alpha=0.9);
gp_axs.plot(xs,ys,'ro', markersize=4*fig_scale)
gp_axs.set_title("Gaussian Process")
#gp_axs.set_xlabel("x")
#gp_axs.set_ylabel("y")
gp_axs.tick_params(axis='both')
gp_axs.set_ylim(ylim)
# Plot Covariance matrix
if show_covariance:
im = axs[1].imshow(C_f, interpolation='none')
axs[1].set_title("Covariance matrix")
fig.colorbar(im);
# Stdev
if show_stdev:
var_f = np.diag(C_f)[:, None]
std_f = np.sqrt(var_f)
gp_axs.fill(np.concatenate([X_test, X_test[::-1]]),
np.concatenate([mu_f+2*std_f,
(mu_f-2*std_f)[::-1]]),
alpha=.5, fc='b', ec='None', label='95% confidence interval')
Gaussian processes#
Model the data as a Gaussian distribution, conditioned on the training points
Show code cell source
@interact
def plot_sin(nr_points=(0,20,1)):
X_sin = np.random.RandomState(seed=3).uniform(0,100,(100,1))
Y_sin = X_sin/100* np.sin(X_sin/5)*3 + np.random.RandomState(seed=0).randn(100,1)*0.5
X_sin_test = np.linspace(0, 100, 100)[:, None]
plot_gp(X_sin,Y_sin,X_sin_test,lengthscale=12, nr_points=nr_points, nr_samples=100, show_covariance=False, ylim=(-7,7))
if not interactive:
plot_sin(nr_points=10)
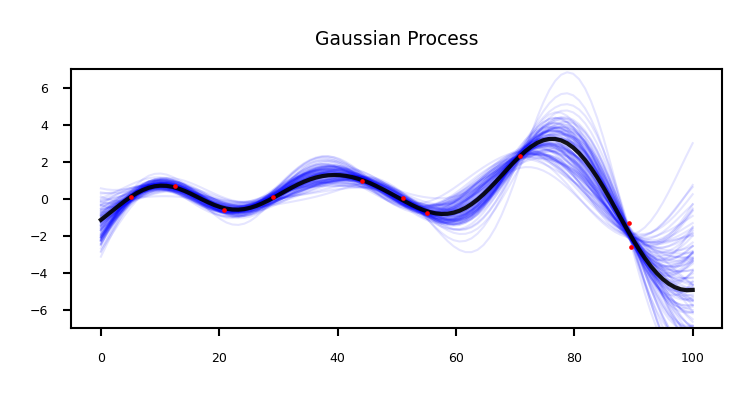
Probabilistic interpretation of regression#
Linear regression (recap):
For one input feature:
We can solve this via linear algebra (closed form solution): \(w^{*} = (X^{T}X)^{-1} X^T Y\)
w = np.linalg.solve(np.dot(X.T, X), np.dot(X.T, y))
\(\mathbf{X}\) is our data matrix with a \(x_0=1\) column to represent the bias \(b\):
Show code cell source
import pods
data = pods.datasets.olympic_marathon_men()
x = data['X']
y = data['Y']
X = np.hstack((np.ones_like(x), x)) # [ones(size(x)) x]
w = np.linalg.solve(np.dot(X.T, X), np.dot(X.T, y))
#print("X: ",X[:5])
#print("xTx: ",np.dot(X.T, X))
#print("xTy: ",np.dot(X.T, y))
Example: Olympic marathon data#
We learned: \( y= w_1 x + w_0 = -0.013 x + 28.895\)
Show code cell source
x_test = np.linspace(1890, 2020, 130)[:, None]
f_test = w[1]*x_test + w[0]
fig = plt.subplots(figsize=(10*fig_scale, 4*fig_scale))
plt.plot(x_test, f_test, 'b-', lw=1)
plt.plot(x, y, 'ro', markersize=4*fig_scale);
plt.title("Linear regression (w: {})".format(w.T))
plt.tick_params(axis='both')
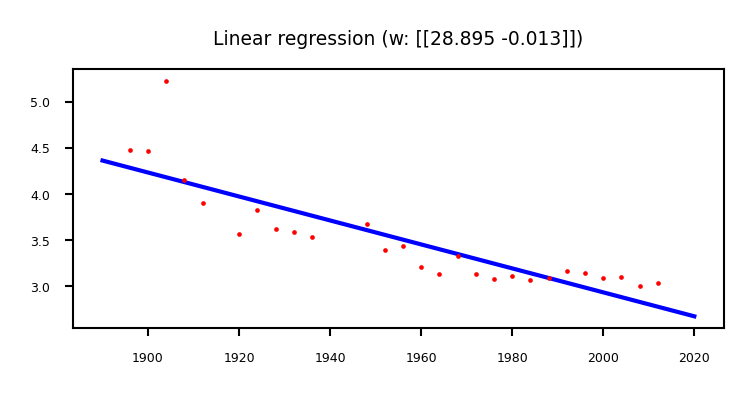
Polynomial regression (recap)#
We can fit a 2nd degree polynomial by using a basis expansion (adding more basis functions):
Show code cell source
Phi = np.hstack([np.ones(x.shape), x, x**2])
w = np.linalg.solve(np.dot(Phi.T, Phi), np.dot(Phi.T, y))
f_test = w[2]*x_test**2 + w[1]*x_test + w[0]
fig = plt.subplots(figsize=(10*fig_scale, 4*fig_scale))
plt.plot(x_test, f_test, 'b-', lw=1)
plt.plot(x, y, 'ro', markersize=4*fig_scale);
plt.title("Polynomial regression (w: {})".format(w.T))
plt.tick_params(axis='both')
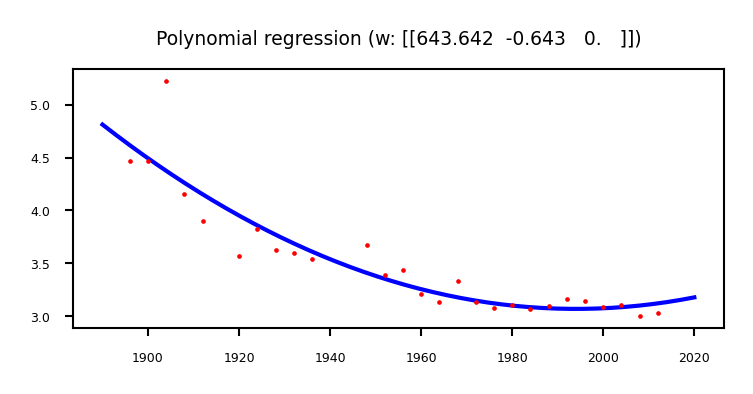
Kernelized regression (recap)#
We can also kernelize the model and learn a dual coefficient per data point
Show code cell source
from sklearn.kernel_ridge import KernelRidge
@interact
def plot_kernel_ridge(poly_degree=(1,8,1), poly_gamma=(-10,-2,1), rbf_gamma=(-10,-2,1), rbf_alpha=(-10,-5,1)):
data = pods.datasets.olympic_marathon_men()
x = data['X']
y = data['Y']
x_test = np.linspace(1890, 2020, 130)[:, None]
plt.rcParams['figure.figsize'] = [6*fig_scale, 4*fig_scale]
reg = KernelRidge(kernel='poly', degree=poly_degree, gamma=np.exp(poly_gamma)).fit(x, y)
plt.plot(x_test, reg.predict(x_test), label="Kernel Ridge (Polynomial)", lw=1, c='b')
reg2 = KernelRidge(kernel='rbf', alpha=np.exp(rbf_alpha), gamma=np.exp(rbf_gamma)).fit(x, y)
plt.plot(x_test, reg2.predict(x_test), label="Kernel Ridge (RBF)", lw=1, c='g')
plt.plot(x, y, 'ro', markersize=4*fig_scale)
plt.title("Kernel Ridge")
plt.tick_params(axis='both')
plt.legend(loc="best");
if not interactive:
plot_kernel_ridge(poly_degree=4, poly_gamma=-6, rbf_gamma=-6, rbf_alpha=-8)
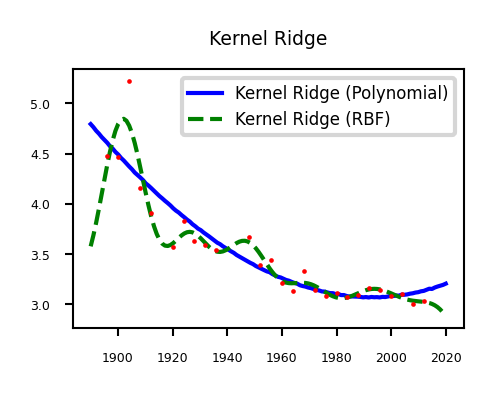
Probabilistic interpretation#
These models do not give us any indication of the (un)certainty of the predictions
Assume that the data is inherently uncertain. This can be modeled explictly by introducing a slack variable, \(\epsilon_i\), known as noise.
Assume that the noise is distributed according to a Gaussian distribution with zero mean and variance \(\sigma^2\).
That means that \(y(x)\) is now a Gaussian distribution with mean \(\mathbf{wx}\) and variance \(\sigma^2\)
We have an uncertainty prediction, but it is the same for all predictions
You would expect to be more certain nearby your training points
Show code cell source
from sklearn.linear_model import BayesianRidge
@interact
def plot_regression(sigma=(0.001,0.01,0.001)):
data = pods.datasets.olympic_marathon_men()
x = data['X']
y = data['Y']
x_test = np.linspace(1890, 2020, 130)[:, None]
ridge = BayesianRidge(alpha_1=1/sigma).fit(x, y)
plt.figure(figsize=(6*fig_scale, 4*fig_scale))
plt.plot(x, y, 'o', c='b', markersize=4*fig_scale)
# We have the fitted sigma but we're ignoring it for now.
y_pred, sigma = ridge.predict(x_test, return_std=True)
plt.fill(np.concatenate([x_test, x_test[::-1]]),
np.concatenate([y_pred - 1.9600 * sigma,
(y_pred + 1.9600 * sigma)[::-1]]),
alpha=.5, fc='b', ec='None', label='95% confidence interval')
plt.plot(x_test, y_pred, 'b-', label='Bayesian Ridge')
plt.tick_params(axis='both')
plt.legend()
Show code cell source
if not interactive:
plot_regression(sigma=0.001)
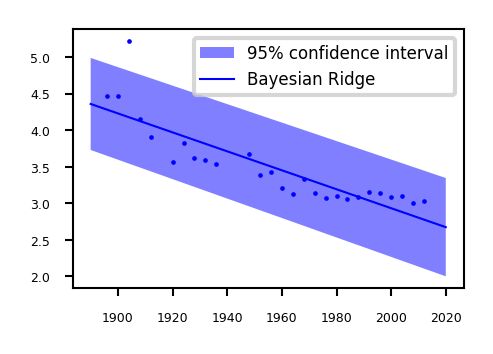
How to learn probabilities?#
Maximum Likelihood Estimation (MLE): Maximize \(P(\textbf{X}|\textbf{w})\)
Corresponds to optimizing \(\mathbf{w}\), using (log) likelihood as the loss function
Every prediction has a mean defined by \(\textbf{w}\) and Gaussian noise
\[P(\textbf{X}|\textbf{w}) = \prod_{i=0}^{n} P(\mathbf{y}_i|\mathbf{x}_i;\mathbf{w}) = \prod_{i=0}^{n} \mathcal{N}(\mathbf{wx,\sigma^2 I})\]
Maximum A Posteriori estimation (MAP): Maximize the posterior \(P(\textbf{w}|\textbf{X})\)
This can be done using Bayes’ rule after we choose a (Gaussian) prior \(P(\textbf{w})\):
\[P(\textbf{w}|\textbf{X}) = \frac{P(\textbf{X}|\textbf{w})P(\textbf{w})}{P(\textbf{X})}\]Bayesian approach: model the prediction \(P(y|x_{test},X)\) directly
Marginalize \(w\) out: consider all possible models (some are more likely)
If prior \(P(\textbf{w})\) is Gaussian, then \(P(y|x_{test},\textbf{X})\) is also Gaussian!
A multivariate Gaussian with mean \(\mu\) and covariance matrix \(\Sigma\)
\[P(y|x_{test},\textbf{X}) = \int_w P(y|x_{test},\textbf{w}) P(\textbf{w}|\textbf{X}) dw = \mathcal{N}(\mathbf{\mu,\Sigma})\]
Gaussian prior \(P(w)\)#
In the Bayesian approach, we assume a prior (Gaussian) distribution for the parameters, \(\mathbf{w} \sim \mathcal{N}(\mathbf{0}, \alpha \mathbf{I})\):
With zero mean (\(\mu\)=0) and covariance matrix \(\alpha \mathbf{I}\). For 2D: \( \alpha \mathbf{I} = \begin{bmatrix} \alpha & 0 \\ 0 & \alpha \end{bmatrix}\)
I.e, \(w_i\) is drawn from a Gaussian density with variance \(\alpha\): \(w_i \sim \mathcal{N}(0,\alpha)\)
Show code cell source
@interact
def interact_prior(alpha=(0.1,1,0.1),contour=False):
# Shape of the joint distribution
mean = [0, 0]
covariance_matrix = np.array([[alpha,0],[0,alpha]])
plot_joint_distribution(mean,covariance_matrix,0,0,contour,labels=['w1','w2'])
Show code cell source
if not interactive:
interact_prior(0.5,contour=False)
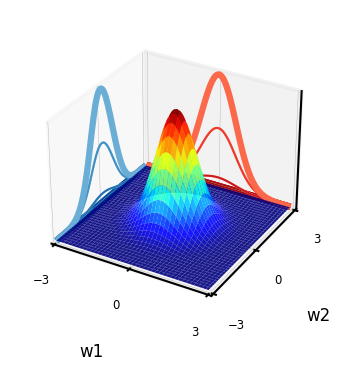
Sampling from the prior (weight space)#
We can sample from the prior distribution to see what form we are imposing on the functions a priori (before seeing any data).
Draw \(w\) (left) independently from a Gaussian density \(\mathbf{w} \sim \mathcal{N}(\mathbf{0}, \alpha\mathbf{I})\)
Use any normally distributed sampling technique, e.g. Box-Mueller transform
Every sample yields a polynomial function \(f(\mathbf{x})\) (right): \(f(\mathbf{x}) = \mathbf{w} \boldsymbol{\phi}(\mathbf{x}).\)
For example, with \(\boldsymbol{\phi}(\mathbf{x})\) being a polynomial:
Show code cell source
alpha = 4. # set prior variance on w
degree = 5 # set the order of the polynomial basis set
sigma2 = 0.01 # set the noise variance
num_pred_data = 100 # how many points to use for plotting predictions
x_pred = np.linspace(1880, 2030, num_pred_data)[:, None] # input locations for predictions
cmap = plt.get_cmap('jet')
# Build the basis matrices (on Olympics data)
def polynomial(x, degree, loc, scale):
degrees = np.arange(degree+1)
return ((x-loc)/scale)**degrees
@interact
def plot_function_space(alpha=(0.1,5,0.5),degree=(1,10,1),random=(0,10,1)):
data = pods.datasets.olympic_marathon_men()
x = data['X']
y = data['Y']
scale = np.max(x) - np.min(x)
loc = np.min(x) + 0.5*scale
x_pred = np.linspace(1880, 2030, num_pred_data)[:, None] # input locations for predictions
Phi_pred = polynomial(x_pred, degree=degree, loc=loc, scale=scale)
Phi = polynomial(x, degree=degree, loc=loc, scale=scale)
fig, axs = plt.subplots(1, 2, figsize=(10*fig_scale, 4*fig_scale))
num_samples = 10
K = degree+1
colors = [cmap(i) for i in np.linspace(0, 1, num_samples)]
for i in range(num_samples):
z_vec = np.random.normal(size=(K, 1))
w_sample = z_vec*np.sqrt(alpha)
f_sample = np.dot(Phi_pred,w_sample)
axs[0].scatter(w_sample[0], w_sample[1], color=colors[i])
axs[0].set(xlabel="w_1", ylabel="w_2")
axs[1].plot(x_pred, f_sample, ls='-', c=colors[i])
axs[0].set_xlim(xmin=-5, xmax=5)
axs[0].set_ylim(ymin=-5, ymax=5)
axs[1].set_ylim(ymin=-5, ymax=5)
axs[0].tick_params(axis='both')
axs[1].tick_params(axis='both')
x1 = np.linspace(-5, 5, 100)
X1, X2 = np.meshgrid(x1, x1)
xy = np.hstack((X1.flatten()[:, None], X2.flatten()[:, None]))
p = stats.multivariate_normal.pdf(xy, mean=[0,0], cov=[[alpha,0],[0,alpha]])
P = p.reshape(len(X1), len(X2))
axs[0].contour(X1, X2, P)
Show code cell source
if not interactive:
plot_function_space(alpha=4, degree=5, random=0)
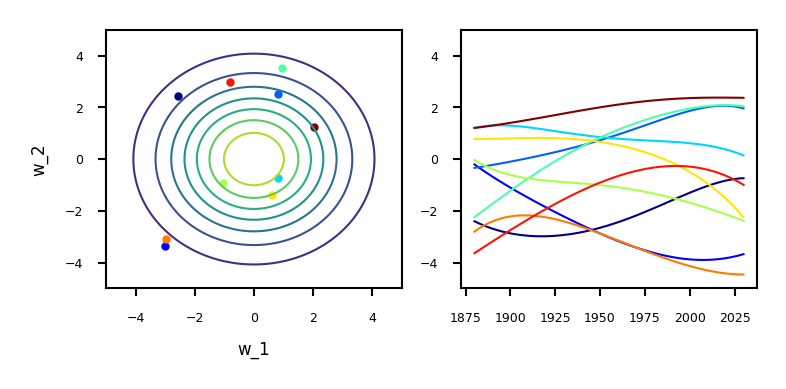
Learning Gaussian distributions#
We assume that our data is Gaussian distributed: \(P(y|x_{test},\textbf{X}) = \mathcal{N}(\mathbf{\mu,\Sigma})\)
Example with learned mean \([m,m]\) and covariance \(\begin{bmatrix} \alpha & \beta \\ \beta & \alpha \end{bmatrix}\)
The blue curve is the predicted \(P(y|x_{test},\textbf{X})\)
Show code cell source
@interact
def interact_prior(x_test=(-3,3,0.5),m=(-0.5,0.5,0.1),alpha=(0.5,1,0.1),beta=(-0.5,0.5,0.1),contour=False):
# Shape of the joint distribution
mean_matrix = [m, m]
covariance_matrix = np.array([[alpha,beta],[beta,alpha]])
plot_joint_distribution(mean_matrix,covariance_matrix,x_test,0,contour,plot_intersect=True,labels=['x_test','y'])
Show code cell source
if not interactive:
fig = plt.figure(figsize=plt.figaspect(0.3)*fig_scale*2)
ax1 = fig.add_subplot(1, 2, 1, projection='3d')
m,alpha,beta = 0,1,0
mean_matrix = [m, m]
covariance_matrix = np.array([[alpha,beta],[beta,alpha]])
ax1.set_title("m=0, alpha=1, beta=0", pad=0)
plot_joint_distribution(mean_matrix,covariance_matrix,0,0,contour=False,labels=['x_test','y'], ax=ax1)
ax2 = fig.add_subplot(1, 2, 2, projection='3d')
m,alpha,beta = 1,0.5,0.3
mean_matrix = [m, m]
covariance_matrix = np.array([[alpha,beta],[beta,alpha]])
ax2.set_title("m=1, alpha=0.5, beta=0.3", pad=0)
plot_joint_distribution(mean_matrix,covariance_matrix,0,0,contour=False, labels=['x_test','y'], ax=ax2)
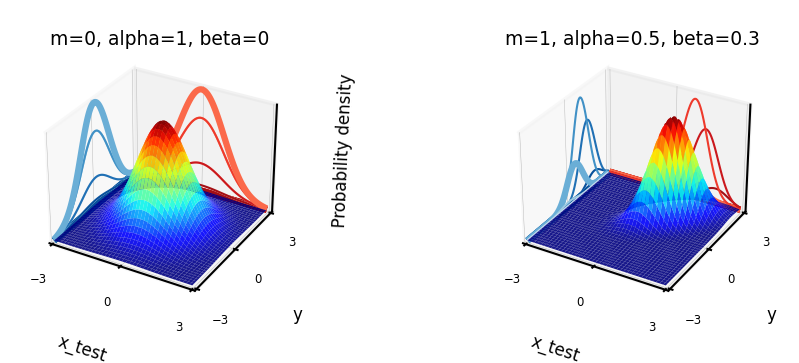
Understanding covariances#
If two variables \(x_i\) covariate strongly, knowing about \(x_1\) tells us a lot about \(x_2\)
If covariance is 0, knowing \(x_1\) tells us nothing about \(x_2\) (the conditional and marginal distributions are the same)
For covariance matrix \(\begin{bmatrix} 1 & \beta \\ \beta & 1 \end{bmatrix}\):
Show code cell source
@interact
def interact_covariance(x1=(-3,3,0.5),x2=(-3,3,0.5),beta=(-0.9,0.9,0.1)):
# Shape of the joint distribution
mean = [0, 0]
covariance_matrix = np.array([[1,beta],[beta,1]])
plot_joint_distribution(mean,covariance_matrix,x1,x2,contour=True, plot_intersect=True, labels=['x1','x2'])
Show code cell source
if not interactive:
fig = plt.figure(figsize=plt.figaspect(0.3)*fig_scale*2)
ax1 = fig.add_subplot(1, 2, 1, projection='3d')
mean = [0, 0]
covariance_matrix = np.array([[1,0],[0,1]])
ax1.set_title("beta=0",fontsize=12*fig_scale, pad=0)
plot_joint_distribution(mean,covariance_matrix,1,1,contour=True, plot_intersect=True, labels=['x1','x2'], ax=ax1)
ax2 = fig.add_subplot(1, 2, 2, projection='3d')
covariance_matrix = np.array([[1,0.9],[0.9,1]])
ax2.set_title("beta=0.9",fontsize=12*fig_scale, pad=0)
plot_joint_distribution(mean,covariance_matrix,1,1,contour=True, plot_intersect=True, labels=['x1','x2'], ax=ax2)
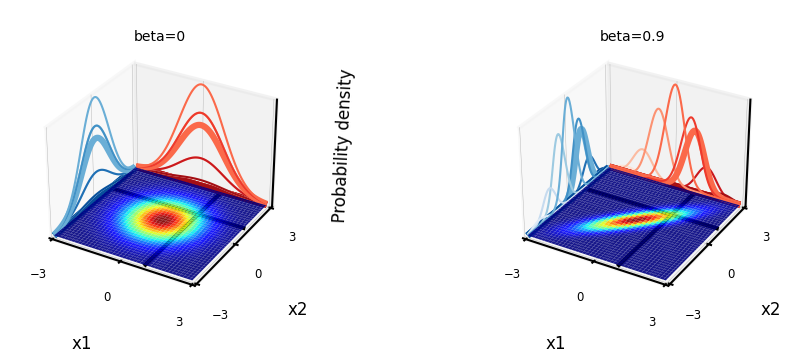
Stochastic processes & sampling from higher-dimensional distributions#
Instead of sampling \(\mathbf{w}\) and then multiplying by \(\boldsymbol{\Phi}\), we can also generate examples of \(f(x)\) directly.
\(\mathbf{f}\) with \(n\) values can be sampled from an \(n\)-dimensional Gaussian distribution with zero mean and covariance matrix \(\mathbf{K} = \alpha \boldsymbol{\Phi}\boldsymbol{\Phi}^\top\):
\(\mathbf{f}\) is a stochastic process: series of normally distributed variables (interpolated in the plot)
Show code cell source
from matplotlib.ticker import MaxNLocator
# Exponentiated quadratic is another name for RBF
def exponentiated_quadratic(x, x_prime, variance, lengthscale):
squared_distance = ((x-x_prime)**2).sum()
return variance*np.exp((-0.5*squared_distance)/lengthscale**2)
# Compute covariances directly
def compute_kernel(X, X2, kernel, **kwargs):
K = np.zeros((X.shape[0], X2.shape[0]))
for i in np.arange(X.shape[0]):
for j in np.arange(X2.shape[0]):
K[i, j] = kernel(X[i, :], X2[j, :], **kwargs)
return K
def plot_process(alpha=1, dimensions=100, num_samples=5, phi="Polynomial", sigma2=0.01, noise=False, length_scale=10.):
x_pred = np.linspace(0, dimensions-1, dimensions)[:, None] # input locations for predictions
if phi == "Identity":
K = alpha*np.eye(dimensions)
elif phi == "RBF":
K = compute_kernel(x_pred, x_pred, exponentiated_quadratic, variance=alpha, lengthscale=length_scale)
else:
if phi == "Polynomial":
degree = 5
elif phi == "Linear":
degree = 1
scale = np.max(x_pred) - np.min(x_pred)
loc = np.min(x_pred) + 0.5*scale
Phi_pred = polynomial(x_pred, degree=degree, loc=loc, scale=scale)
K = alpha*np.dot(Phi_pred, Phi_pred.T)
if noise:
K += sigma2*np.eye(x_pred.size)
if dimensions==2:
fig, axs = plt.subplots(1, 3, figsize=(10*fig_scale, 4*fig_scale))
ax_d, ax_s, ax_c = axs[0], axs[1], axs[2]
else:
fig, axs = plt.subplots(1, 2, figsize=(10*fig_scale, 4*fig_scale))
ax_s, ax_c = axs[0], axs[1]
im = ax_c.imshow(K, interpolation='none')
ax_c.set_title("Covariance matrix")
ax_c.tick_params(axis='both')
ax_c.xaxis.set_major_locator(MaxNLocator(integer=True))
ax_c.yaxis.set_major_locator(MaxNLocator(integer=True))
ax_c.tick_params(axis='both', pad=0)
fig.colorbar(im);
samples = np.random.RandomState(seed=4).multivariate_normal(np.zeros(dimensions), K, num_samples).T;
colors = [cmap(i) for i in np.linspace(0, 1, num_samples)]
for i in range(samples.shape[1]):
ax_s.plot(x_pred, samples[:,i], color=colors[i], ls='-');
ax_s.xaxis.set_major_locator(MaxNLocator(integer=True))
ax_s.set_title("Interpolated samples \n from Gaussian")
ax_s.set_xlabel("X")
ax_s.tick_params(axis='both')
ax_s.set_aspect(1./ax_s.get_data_ratio())
ax_s.tick_params(axis='both', pad=0)
if dimensions==2:
ax_d.scatter(samples[0],samples[1], color=colors)
x1 = np.linspace(-2.5, 2.5, 100)
X1, X2 = np.meshgrid(x1, x1)
xy = np.hstack((X1.flatten()[:, None], X2.flatten()[:, None]))
p = stats.multivariate_normal.pdf(xy, mean=[0,0], cov=K)
P = p.reshape(len(X1), len(X2))
ax_d.contour(X1, X2, P)
ax_d.tick_params(axis='both')
ax_d.set_aspect(1./ax_d.get_data_ratio())
ax_d.set_xlabel("x0")
ax_d.set_ylabel("x1")
ax_d.xaxis.set_major_locator(MaxNLocator(integer=True))
ax_d.yaxis.set_major_locator(MaxNLocator(integer=True))
ax_d.set_title("Samples from 2D Gaussian")
ax_d.tick_params(axis='both', pad=0)
@interact
def plot_process_noiseless(alpha=(0.1,2,0.1), dimensions=(2,100,1), phi=["Identity","Linear","Polynomial"]):
plot_process(alpha=alpha, dimensions=dimensions, phi=phi, noise=False)
Show code cell source
if not interactive:
plot_process(alpha=1, dimensions=2, phi="Polynomial")
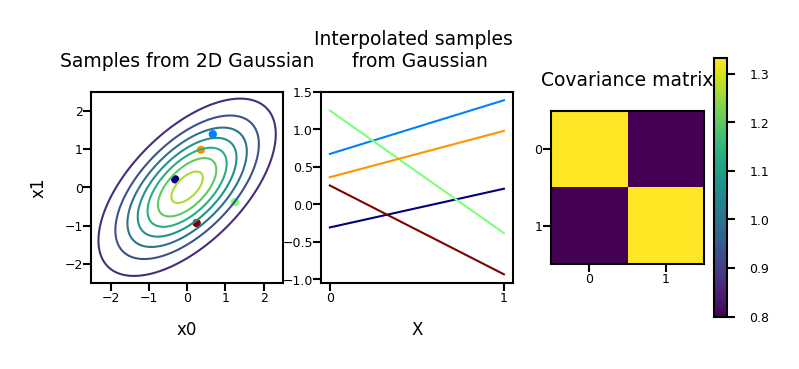
Repeat for 40 dimensions, with \(\boldsymbol{\Phi}\) the polynomial transform:
Show code cell source
plot_process(alpha=1, dimensions=40, phi="Polynomial")
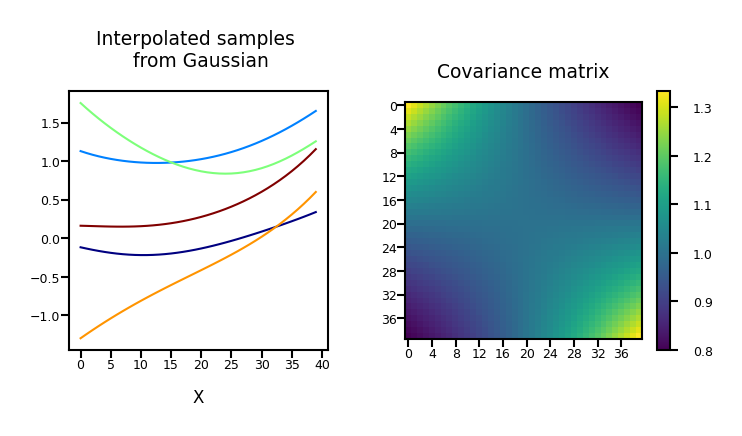
Noisy functions#
We normally add Gaussian noise to obtain our observations:
Show code cell source
@interact
def plot_covm_noise(sigma=(0.01,0.1,0.01)):
plot_process(alpha=1, dimensions=100, phi="Polynomial", sigma2=sigma, noise=True)
Show code cell source
if not interactive:
plot_process(alpha=1, dimensions=100, phi="Polynomial", sigma2=0.02, noise=True)
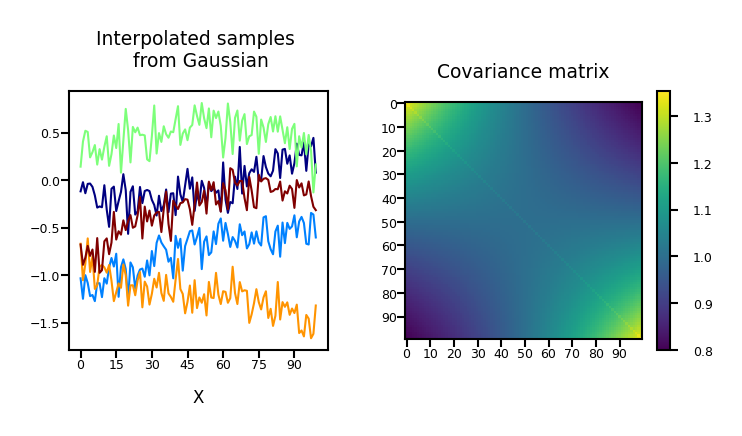
Gaussian Process#
Usually, we want our functions to be smooth: if two points are similar/nearby, the predictions should be similar.
Hence, we need a similarity measure (a kernel)
In a Gaussian process we can do this by specifying the covariance function directly (not as \(\mathbf{K} = \alpha \boldsymbol{\Phi}\boldsymbol{\Phi}^\top\))
The covariance matrix is simply the kernel matrix: \(\mathbf{f} \sim \mathcal{N}(\mathbf{0},\mathbf{K})\)
The RBF (Gaussian) covariance function (or kernel) is specified by
where \(\left\Vert\mathbf{x} - \mathbf{x}^\prime\right\Vert^2\) is the squared distance between the two input vectors
and the length parameter \(l\) controls the smoothness of the function and \(\alpha\) the vertical variation.
Now the influence of a point decreases smoothly but exponentially
These are our priors \(P(y) = \mathcal{N}(\mathbf{0,\mathbf{K}})\), with mean 0
We now want to condition it on our training data: \(P(y|x_{test},\textbf{X}) = \mathcal{N}(\mathbf{\mu,\Sigma})\)
Show code cell source
@interact
def plot_gprocess(alpha=(0.1,2,0.1), lengthscale=(1,20,1), dimensions=(2,100,1), nr_samples=(1,21,5)):
plot_process(alpha=alpha, dimensions=dimensions, phi="RBF", length_scale=lengthscale, num_samples=nr_samples)
if not interactive:
plot_gprocess(alpha=1, lengthscale=10, dimensions=100, nr_samples=12)
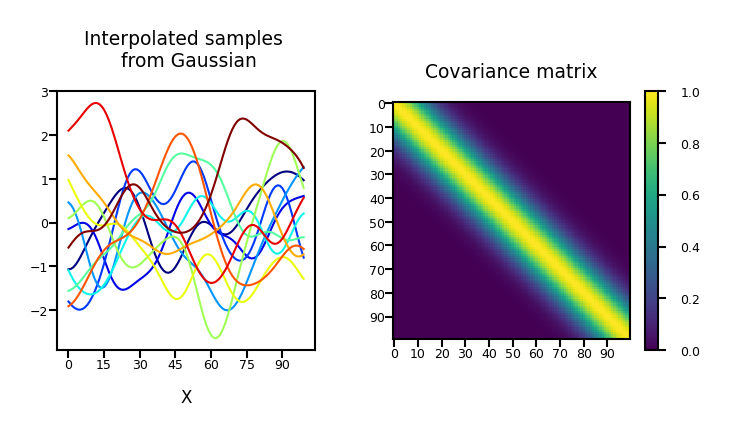
Computing the posterior \(P(\mathbf{y}|\mathbf{X})\)#
Assuming that \(P(X)\) is a Gaussian density with a covariance given by kernel matrix \(\mathbf{K}\), the model likelihood becomes:
Hence, the negative log likelihood (the objective function) is given by:
The model parameters (e.g. noise variance \(\sigma^2\)) and the kernel parameters (e.g. lengthscale, variance) can be embedded in the covariance function and learned from data.
Good news: This loss function can be optimized using linear algebra (Cholesky Decomposition)
Bad news: This is cubic in the number of data points AND the number of features: \(\mathcal{O}(n^3 d^3)\)
class GP():
def __init__(self, X, y, sigma2, kernel, **kwargs):
self.K = compute_kernel(X, X, kernel, **kwargs)
self.X = X
self.y = y
self.sigma2 = sigma2
self.kernel = kernel
self.kernel_args = kwargs
self.update_inverse()
def update_inverse(self):
# Precompute the inverse covariance and some quantities of interest
## NOTE: Not the correct *numerical* way to compute this! For ease of use.
self.Kinv = np.linalg.inv(self.K+self.sigma2*np.eye(self.K.shape[0]))
# the log determinant of the covariance matrix.
self.logdetK = np.linalg.det(self.K+self.sigma2*np.eye(self.K.shape[0]))
# The matrix inner product of the inverse covariance
self.Kinvy = np.dot(self.Kinv, self.y)
self.yKinvy = (self.y*self.Kinvy).sum()
def log_likelihood(self):
# use the pre-computes to return the likelihood
return -0.5*(self.K.shape[0]*np.log(2*np.pi) + self.logdetK + self.yKinvy)
def objective(self):
# use the pre-computes to return the objective function
return -self.log_likelihood()
Making predictions#
The model makes predictions for \(\mathbf{f}\) that are unaffected by future values of \(\mathbf{f}^*\).
If we think of \(\mathbf{f}^*\) as test points, we can still write down a joint probability density over the training observations, \(\mathbf{f}\) and the test observations, \(\mathbf{f}^*\).
This joint probability density will be Gaussian, with a covariance matrix given by our kernel function, \(k(\mathbf{x}_i, \mathbf{x}_j)\). $\( \begin{bmatrix}\mathbf{f} \\ \mathbf{f}^*\end{bmatrix} \sim \mathcal{N}\left(\mathbf{0}, \begin{bmatrix} \mathbf{K} & \mathbf{K}_\ast \\ \mathbf{K}_\ast^\top & \mathbf{K}_{\ast,\ast}\end{bmatrix}\right) \)$
where \(\mathbf{K}\) is the kernel matrix computed between all the training points,
\(\mathbf{K}_\ast\) is the kernel matrix computed between the training points and the test points,
\(\mathbf{K}_{\ast,\ast}\) is the kernel matrix computed between all the tests points and themselves.
Analogy
The joint probability of two variable \(x_1\) and \(x_2\) following a multivariate Gaussian is: $\( \begin{bmatrix}x_1 \\ x_2 \end{bmatrix} \sim \mathcal{N}\left(\mathbf{0}, \begin{bmatrix} \mathbf{K} & \mathbf{K}_\ast \\ \mathbf{K}_\ast^\top & \mathbf{K}_{\ast,\ast}\end{bmatrix}\right) \)$
Conditional Density \(P(\mathbf{y}|x_{test} , \mathbf{X})\)#
Finally, we need to define conditional distributions to answer particular questions of interest
We will need the conditional density for making predictions.
with a mean given by \( \boldsymbol{\mu}_f = \mathbf{K}_*^\top \left[\mathbf{K} + \sigma^2 \mathbf{I}\right]^{-1} \mathbf{y} \)
and a covariance given by \( \mathbf{C}_f = \mathbf{K}_{*,*} - \mathbf{K}_*^\top \left[\mathbf{K} + \sigma^2 \mathbf{I}\right]^{-1} \mathbf{K}_\ast. \)
Show code cell source
model = GP(x, y, sigma2, exponentiated_quadratic, variance=variance, lengthscale=lengthscale)
mu_f, C_f = model.posterior_f(x_pred, y)
Gaussian process example#
We can now get the mean and covariance learned on our dataset, and sample from this distribution!
Show code cell source
def shuffled_olympics():
data = pods.datasets.olympic_marathon_men()
X = data['X']
Y = data['Y']
perm = np.random.RandomState(seed=0).permutation(len(X))
x_shuffle, y_shuffle = X[perm], Y[perm]
X_test = np.linspace(1890, 2020, 100)[:, None]
return x_shuffle, y_shuffle, X_test
@interact
def plot_gp_olympics(nr_points=(0,27,1)):
x, y, xt = shuffled_olympics()
plot_gp(x, y, xt, lengthscale=12, nr_points=nr_points, nr_samples=100, show_covariance=True, ylim=(-4,8))
if not interactive:
plot_gp_olympics(nr_points=13)
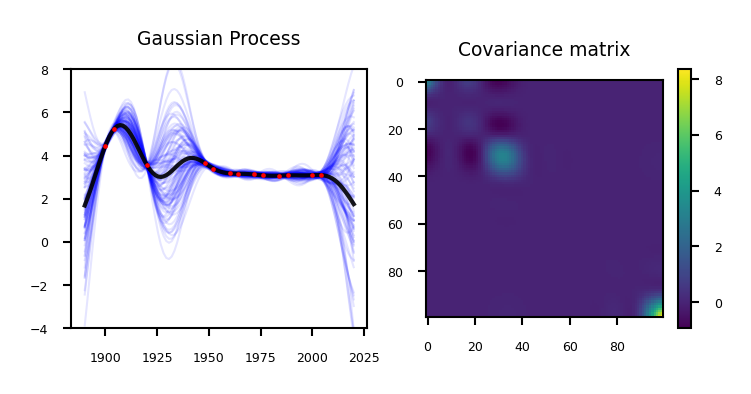
Remember that our prediction is the sum of the mean and the variance: \(P(\mathbf{y}|x_{test} , \mathbf{X}) = \mathcal{N}(\mathbf{\mu,\Sigma})\)
The mean is the same as the one computed with kernel ridge (if given the same kernel and hyperparameters)
The Gaussian process learned the covariance and the hyperparameters
Show code cell source
@interact
def plot_gp_olympics_mean(nr_points=(0,27,1), add_mean=False):
x, y, xt = shuffled_olympics()
plot_gp(x, y, xt, lengthscale=12, nr_points=nr_points, add_mean=add_mean, nr_samples=100, show_covariance=True, ylim=(-4,8))
if not interactive:
plot_gp_olympics_mean(nr_points=13)
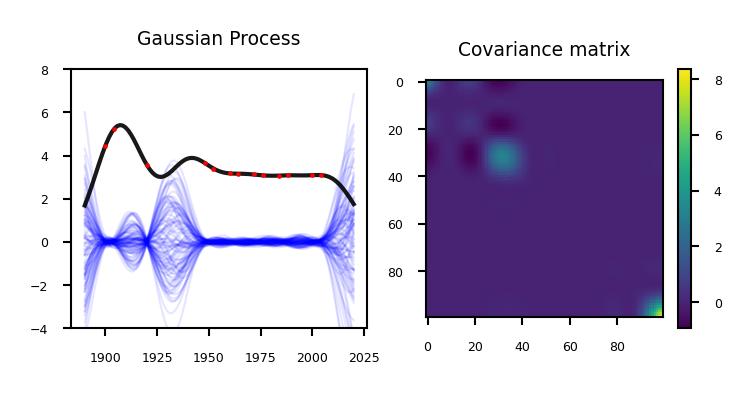
The values on the diagonal of the covariance matrix give us the variance, so we can simply plot the mean and 95% confidence interval
Show code cell source
@interact
def plot_gp_olympics_stdev(nr_points=(0,27,1), lengthscale=(4,20,1)):
x, y, xt = shuffled_olympics()
plot_gp(x, y, xt, lengthscale=lengthscale, nr_points=nr_points, nr_samples=100, show_covariance=True, show_stdev=True, ylim=(-4,8))
if not interactive:
plot_gp_olympics_stdev(nr_points=13, lengthscale=12)
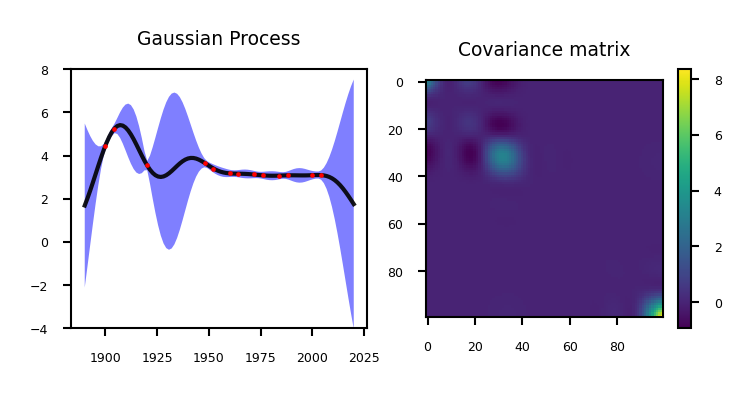
Gaussian Processes in practice (with GPy)#
GPyRegression
Generate a kernel first
State the dimensionality of your input data
Variance and lengthscale are optional, default = 1
kernel = GPy.kern.RBF(input_dim=1, variance=1., lengthscale=1.)
Other kernels:
GPy.kern.BasisFuncKernel?
Build model:
m = GPy.models.GPRegression(X,Y,kernel)
Show code cell source
import GPy
custom_kernel = GPy.kern.RBF(1) + GPy.kern.Linear(1) * GPy.kern.RatQuad(1.0)
figure, axes = plt.subplots(3,4, figsize=(10*fig_scale,7*fig_scale), tight_layout=True)
kerns = [GPy.kern.RBF(1), GPy.kern.Exponential(1), GPy.kern.Matern32(1),
GPy.kern.Matern52(1), GPy.kern.Brownian(1),GPy.kern.RatQuad(1),
GPy.kern.Linear(1), GPy.kern.PeriodicExponential(1), GPy.kern.White(1),
GPy.kern.Poly(1), GPy.kern.MLP(1), custom_kernel]
for k,a in zip(kerns, axes.flatten()):
k.plot(ax=a, x=0.01)
a.set_title(k.name.replace('_', ' '))
a.tick_params(axis='both')
a.set_ylabel("")
a.set_xlabel("")
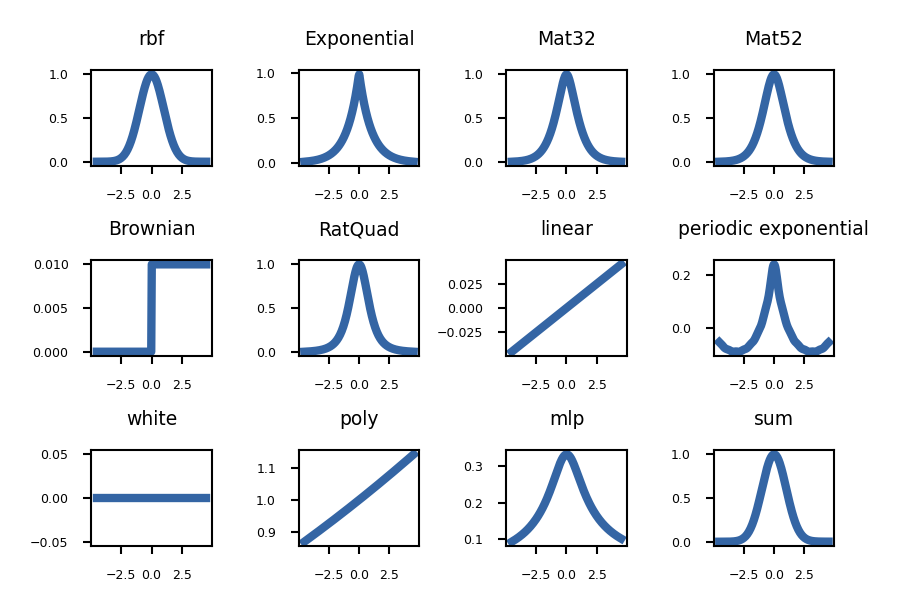
Matern
is a generalized RBF kernel that can scale between RBF and Exponential. Sum
is RBF + Linear * RatQuad
Effect of different kernels#
#!pip install "numpy<=1.23.5"
# Needed because of an unfixed deprecation bug in GPy
import copy
np.random.seed(5)
Xr = np.random.uniform(-5.,5.,(10,1))
Yr = Xr + np.sin(Xr*2) + np.random.randn(10,1)*0.05
# I need to make a deep copy since I will be training the kernels
kerneldict = {k.name.replace('_', ' ') : k for k in copy.deepcopy(kerns)}
height_ratio=1
@interact
def plot_kernels(kernel=kerneldict.keys()):
plt.rcParams.update({"lines.markersize": 4})
figure, axes = plt.subplots(1,2, figsize=(8*fig_scale,5*fig_scale*height_ratio), tight_layout=True, gridspec_kw={'width_ratios': [1, 3]})
kerneldict[kernel].plot(ax=axes[0], x=0.01)
m = GPy.models.GPRegression(Xr,Yr,kerneldict[kernel])
m.optimize_restarts(num_restarts = 3, verbose=0)
fig = m.plot(ax=axes[1])
axes[0].set_title(kernel)
axes[0].get_xaxis().set_visible(False)
axes[0].get_yaxis().set_visible(False)
if not interactive:
height_ratio=0.6
plot_kernels("rbf")
plot_kernels("sum")
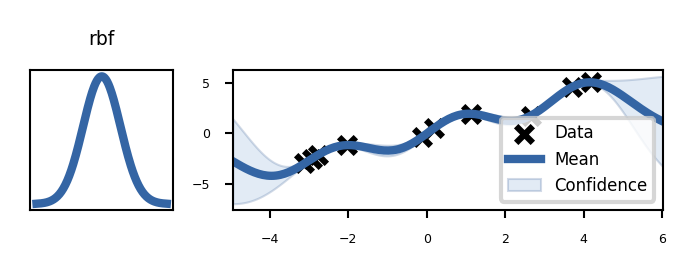
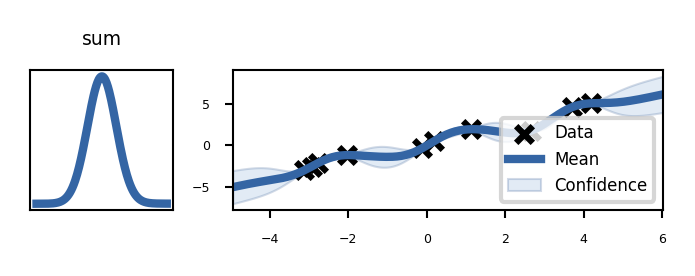
Build the untrained GP. The shaded region corresponds to ~95% confidence intervals (i.e. +/- 2 standard deviation)
Show code cell source
# Generate noisy sine data
X = np.random.uniform(-5.,5.,(10,1))
Y = np.sin(X) + np.random.randn(10,1)*0.05
# Build untrained model
kernel = GPy.kern.RBF(input_dim=1, variance=1., lengthscale=1.)
m = GPy.models.GPRegression(X,Y,kernel)
fig = m.plot()
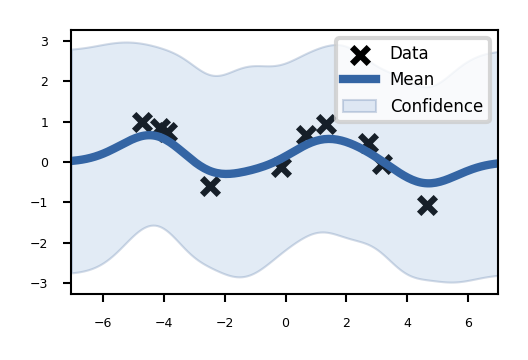
Train the model (optimize the kernel parameters): maximize the likelihood of the data.
Best to optimize with a few restarts: the optimizer may converges to the high-noise solution. The optimizer is then restarted with a few random initializations of the parameter values.
Show code cell source
m.optimize_restarts(num_restarts = 3, verbose=0)
fig = m.plot()
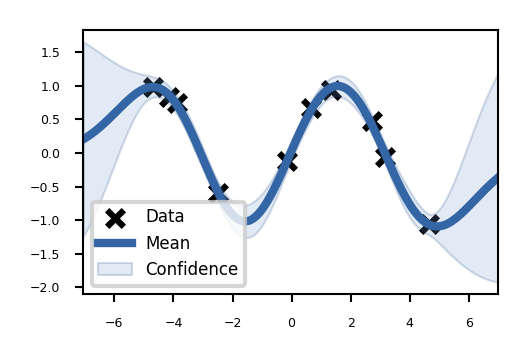
You can also show results in 2D
Show code cell source
# sample inputs and outputs
X = np.random.uniform(-3.,3.,(50,2))
Y = np.sin(X[:,0:1]) * np.sin(X[:,1:2])+np.random.randn(50,1)*0.05
ker = GPy.kern.Matern52(2,ARD=True) + GPy.kern.White(2)
# create simple GP model
m = GPy.models.GPRegression(X,Y,ker)
# optimize and plot
m.optimize(max_f_eval = 1000)
fig = m.plot()
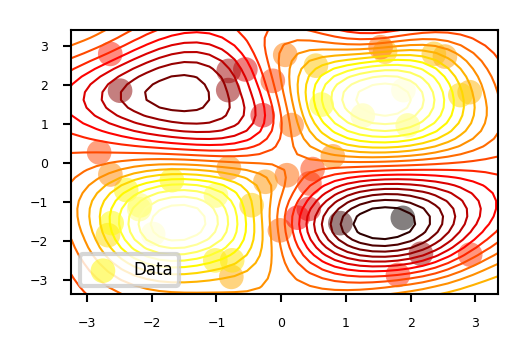
We can plot 2D slices using the fixed_inputs
argument to the plot function.
fixed_inputs
is a list of tuples containing which of the inputs to fix, and to which value.
Show code cell source
slices = [-1, 0, 1.5]
figure = GPy.plotting.plotting_library().figure(3, 1)
figure.set_size_inches(8*fig_scale,5*fig_scale)
for i, y in zip(range(3), slices):
# Use fixed_inputs=[(0,y)] for vertical slices
canvas = m.plot(figure=figure, fixed_inputs=[(1,y)], row=(i+1), plot_data=False)
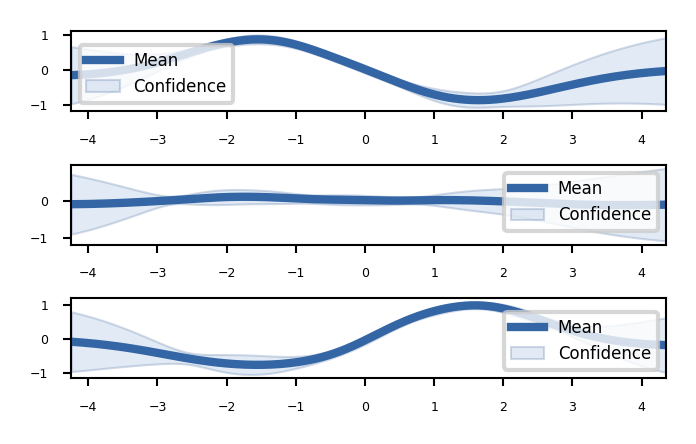
Gaussian Processes with scikit-learn#
GaussianProcessRegressor
Hyperparameters:
kernel
: kernel specifying the covariance function of the GPDefault: “1.0 * RBF(1.0)”
Typically leave at default. Will be optimized during fitting
alpha
: regularization parameterTikhonov regularization of covariance between the training points.
Adds a (small) value to diagonal of the kernel matrix during fitting.
Larger values:
correspond to increased noise level in the observations
also reduce potential numerical issues during fitting
Default: 1e-10
n_restarts_optimizer
: number of restarts of the optimizerDefault: 0. Best to do at least a few iterations.
Optimizer finds kernel parameters maximizing log-marginal likelihood
Retrieve predictions and confidence interval after fitting:
y_pred, sigma = gp.predict(x, return_std=True)
Example
Show code cell source
from sklearn.gaussian_process import GaussianProcessRegressor
from sklearn.gaussian_process.kernels import RBF, ConstantKernel as C
def f(x):
"""The function to predict."""
return x * np.sin(x)
X = np.atleast_2d([1., 3., 5., 6., 7., 8.]).T
# Observations
y = f(X).ravel()
# Mesh the input space for evaluations of the real function, the prediction and
# its MSE
x = np.atleast_2d(np.linspace(0, 10, 1000)).T
# Instanciate a Gaussian Process model
kernel = C(1.0, (1e-3, 1e3)) * RBF(10, (1e-2, 1e2))
gp = GaussianProcessRegressor(kernel=kernel, n_restarts_optimizer=9)
# Fit to data using Maximum Likelihood Estimation of the parameters
gp.fit(X, y)
# Make the prediction on the meshed x-axis (ask for MSE as well)
y_pred, sigma = gp.predict(x, return_std=True)
# Plot the function, the prediction and the 95% confidence interval based on
# the MSE
fig = plt.figure()
plt.plot(x, f(x), 'r:', label=u'$f(x) = x\,\sin(x)$')
plt.plot(X, y, 'r.', markersize=2, label=u'Observations')
plt.plot(x, y_pred, 'b-', label=u'Prediction')
plt.fill(np.concatenate([x, x[::-1]]),
np.concatenate([y_pred - 1.9600 * sigma,
(y_pred + 1.9600 * sigma)[::-1]]),
alpha=.5, fc='b', ec='None', label='95% confidence interval')
plt.xlabel('$x$')
plt.ylabel('$f(x)$')
plt.ylim(-10, 20)
plt.legend(loc='upper left');
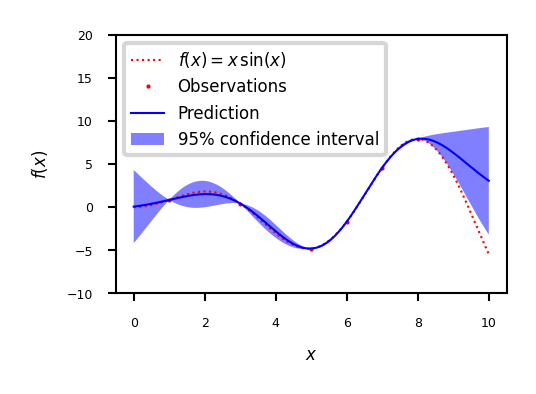
Example with noisy data
Show code cell source
X = np.linspace(0.1, 9.9, 20)
X = np.atleast_2d(X).T
# Observations and noise
y = f(X).ravel()
dy = 0.5 + 1.0 * np.random.random(y.shape)
noise = np.random.normal(0, dy)
y += noise
# Instanciate a Gaussian Process model
gp = GaussianProcessRegressor(kernel=kernel, alpha=(dy / y) ** 2,
n_restarts_optimizer=10)
# Fit to data using Maximum Likelihood Estimation of the parameters
gp.fit(X, y)
# Make the prediction on the meshed x-axis (ask for MSE as well)
y_pred, sigma = gp.predict(x, return_std=True)
# Plot the function, the prediction and the 95% confidence interval based on
# the MSE
fig = plt.figure()
plt.plot(x, f(x), 'r:', label=u'$f(x) = x\,\sin(x)$')
plt.errorbar(X.ravel(), y, dy, fmt='r.', markersize=2, label=u'Observations')
plt.plot(x, y_pred, 'b-', label=u'Prediction')
plt.fill(np.concatenate([x, x[::-1]]),
np.concatenate([y_pred - 1.9600 * sigma,
(y_pred + 1.9600 * sigma)[::-1]]),
alpha=.5, fc='b', ec='None', label='95% confidence interval')
plt.xlabel('$x$')
plt.ylabel('$f(x)$')
plt.ylim(-10, 20)
plt.legend(loc='upper left')
plt.show()
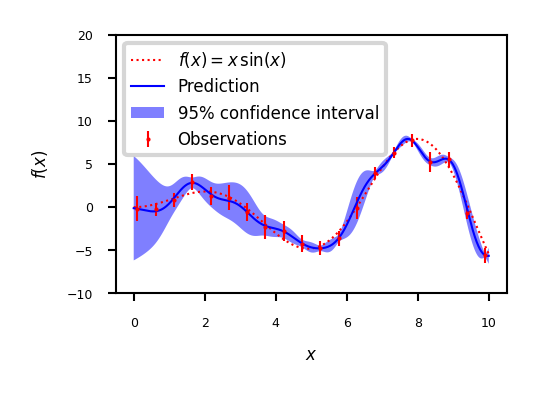
Gaussian processes: Conclusions#
Advantages:
The prediction is probabilistic (Gaussian) so that one can compute empirical confidence intervals.
The prediction interpolates the observations (at least for regular kernels).
Versatile: different kernels can be specified.
Disadvantages:
They are typically not sparse, i.e., they use the whole sample/feature information to perform the prediction.
Sparse GPs also exist: they remember only the most important points
They lose efficiency in high dimensional spaces – namely when the number of features exceeds a few dozens.
Gaussian processes and neural networks#
You can prove that a Gaussian process is equivalent to a neural network with one layer and an infinite number of nodes
You can build deep Gaussian Processes by constructing layers of GPs
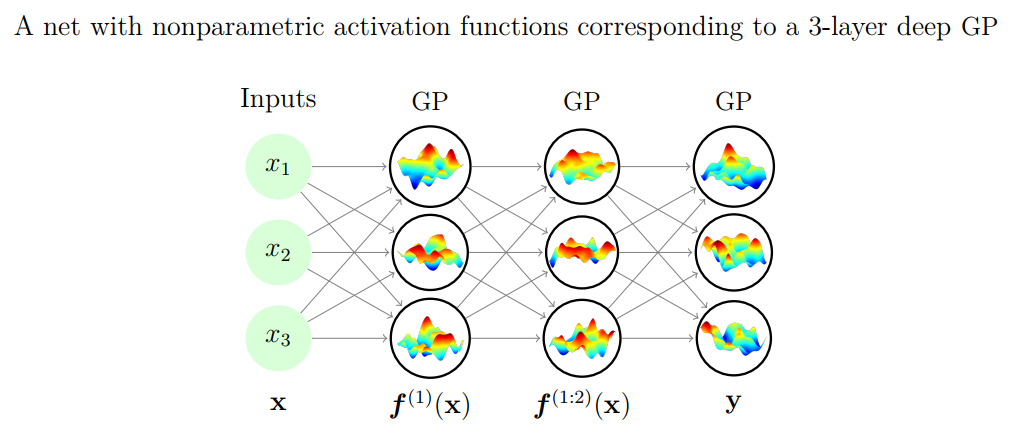
Bayesian optimization#
The incremental updates you can do with Bayesian models allow a more effective way to optimize functions
E.g. to optimize the hyperparameter settings of a machine learning algorithm/pipeline
After a number of random search iterations we know more about the performance of hyperparameter settings on the given dataset
We can use this data to train a model, and predict which other hyperparameter values might be useful
More generally, this is called model-based optimization
This model is called a surrogate model
This is often a probabilistic (e.g. Bayesian) model that predicts confidence intervals for all hyperparameter settings
We use the predictions of this model to choose the next point to evaluate
With every new evaluation, we update the surrogate model and repeat
Example (see figure):#
Consider only 1 continuous hyperparameter (X-axis)
You can also do this for many more hyperparameters
Y-axis shows cross-validation performance
Evaluate a number of random hyperparameter settings (black dots)
Sometimes an initialization design is used
Train a model, and predict the expected performance of other (unseen) hyperparameter values
Mean value (black line) and distribution (blue band)
An acquisition function (green line) trades off maximal expected performace and maximal uncertainty
Exploitation vs exploration
Optimal value of the asquisition function is the next hyperparameter setting to be evaluated
Repeat a fixed number of times, or until time budget runs out
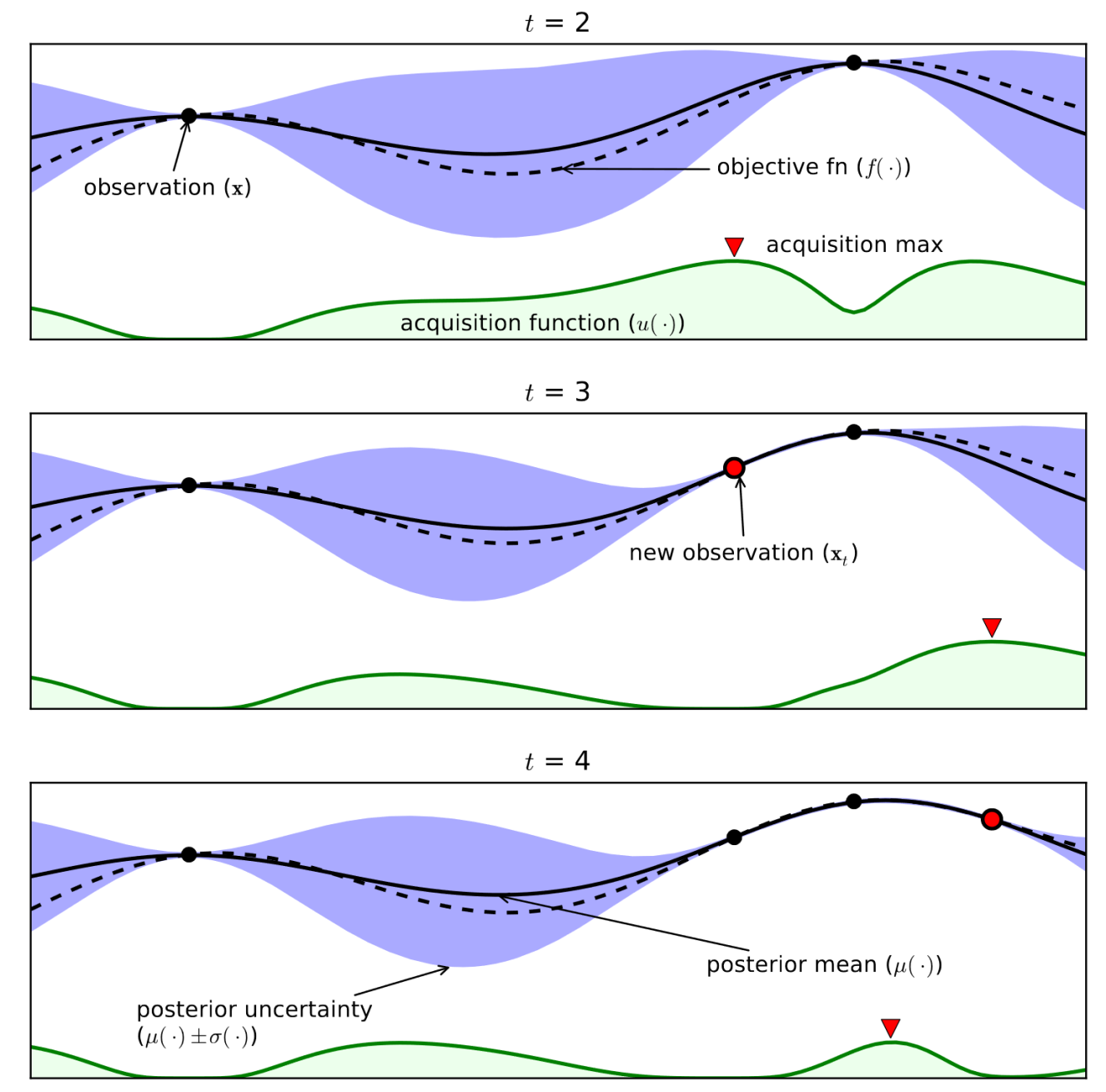
Shahriari et al. Taking the Human Out of the Loop: A Review of Bayesian Optimization
In 2 dimensions:
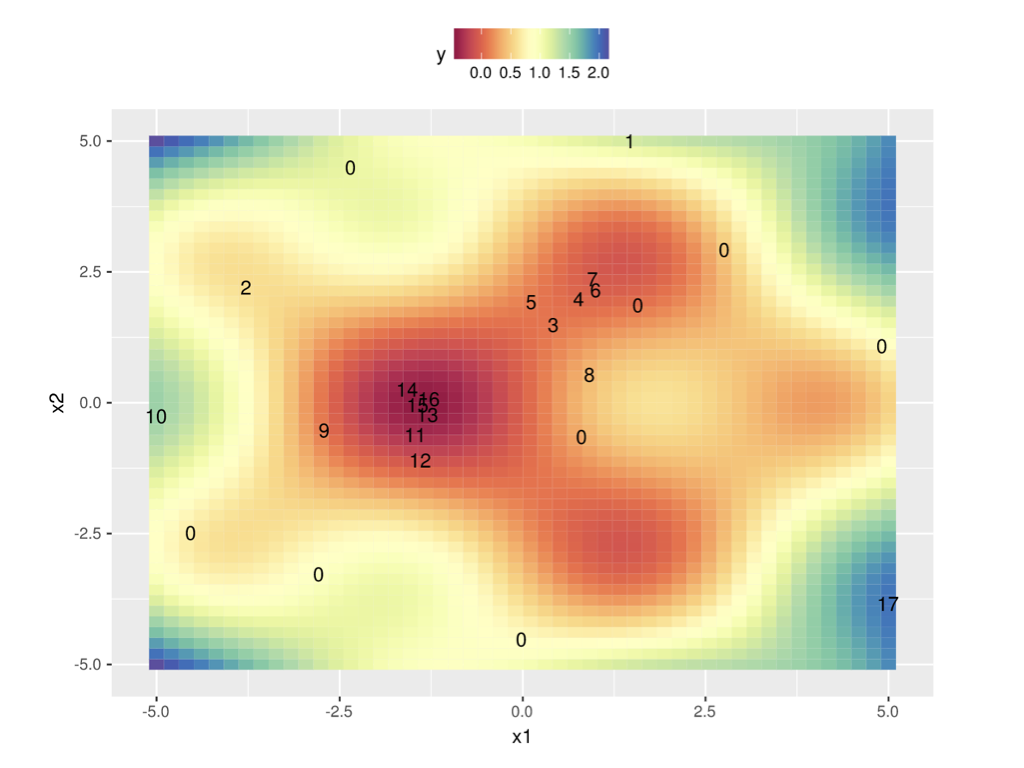
Surrogate models#
Surrogate model can be anything as long as it can do regression and is probabilistic
Gaussian Processes are commonly used
Smooth, good extrapolation, but don’t scale well to many hyperparameters (cubic)
Sparse GPs: select ‘inducing points’ that minimize info loss, more scalable
Multi-task GPs: transfer surrogate models from other tasks
Random Forests
A lot more scalable, but don’t extrapolate well
Often an interpolation between predictions is used instead of the raw (step-wise) predictions
Bayesian Neural Networks:
Expensive, sensitive to hyperparameters
Acquisition Functions#
When we have trained the surrogate model, we ask it to predict a number of samples
Can be simply random sampling
Better: Thompson sampling
fit a Gaussian distribution (a mixture of Gaussians) over the sampled points
sample new points close to the means of the fitted Gaussians
Typical acquisition function: Expected Improvement
Models the predicted performance as a Gaussian distribution with the predicted mean and standard deviation
Computes the expected performance improvement over the previous best configuration \(\mathbf{X^+}\): $\(EI(X) := \mathbb{E}\left[ \max\{0, f(\mathbf{X^+}) - f_{t+1}(\mathbf{X}) \} \right]\)$
Computing the expected performance requires an integration over the posterior distribution, but has a closed form solution.
Bayesian Optimization: conclusions#
More efficient way to optimize hyperparameters
More similar to what humans would do
Harder to parallellize
Choice of surrogate model depends on your search space
Very active research area
For very high-dimensional search spaces, random forests are popular